Redis 是一款高性能的键值数据库,其支持多种数据类型(String、Hash、List、Set、ZSet、Geo)。在开发中,经常会遇到需要插入大量数据的场景。如果逐条插入,性能会显得较低,而采用 Pipeline 批量插入 能大幅提升插入效率。本文将基于常见的 Redis 数据类型,对比循环插入与批量插入的性能差异。 以下测试结果仅供参考,真实环境请多测试!
1. 测试环境说明
- Redis 版本:3.x、5.x 或更高
- Spring Boot 环境:
spring-boot-starter-data-redis
测试过程中,我们将模拟插入 1 万 / 10 万条数据,并记录执行时间。以下是常见的 Redis 数据类型及其插入方法。
2. 各数据类型的插入实现
2.1 String 类型
逐条使用 opsForValue().set()
方法进行插入:
/**
* String类型测试循环插入性能
* @param dataCount
*/
public void testInsertPerformanceStr(int dataCount) {
long beginTime = System.currentTimeMillis();
for (int i = 0; i < dataCount; i++) {
stringRedisTemplate.opsForValue().set(StrKey+i, "value" + i);
}
log.info("使用String类型循环插入{}条数据,总耗时为: {} ms",dataCount, System.currentTimeMillis() - beginTime);
}
通过 Redis Pipeline
批量操作提升性能:
/**
* String类型测试批量处理插入性能
*
* @param dataCount 插入的数据总量
*/
public void testBatchInsertOptimizedStr(int dataCount) {
// 开始计时
long startTime = System.currentTimeMillis();
// 使用 Redis Pipeline 进行批量插入
stringRedisTemplate.executePipelined((RedisCallback<Object>) connection -> {
for (int i = 0; i < dataCount; i++) {
// 构造键值对
String key = StrKey + i;
String value = "value" + i;
// 将命令加入 pipeline
connection.stringCommands().set(key.getBytes(), value.getBytes());
}
return null;
});
// 结束计时
long elapsedTime = System.currentTimeMillis() - startTime;
log.info("使用String类型批量插入{}条数据,总耗时为: {}ms", dataCount, elapsedTime);
使用 Lua 脚本删除匹配的键
//String luaScript = "local keys = redis.call('keys', ARGV[1]) " +
// "for i, key in ipairs(keys) do " +
// "redis.call('del', key) " +
// "end " +
// "return #keys";
//DefaultRedisScript<Long> script = new DefaultRedisScript<>(luaScript, Long.class);
//Long deletedKeysCount = redisTemplate.execute(script, Collections.emptyList(), StrKey + "*");
//
//log.info("成功删除前缀为 {} 的所有键,共删除 {} 个键", StrKey, deletedKeysCount);
}
性能对比
插入方式 | 插入数据量 | 耗时(ms) |
---|
循环插入 | 1万条 | 500+ |
批量插入 | 1 万条 | 150+ |
2.2 ZSet(有序集合)类型
/**
* ZSet测试循环插入性能
*
* @param dataCount
*/
public void testInsertPerformanceZSet(int dataCount) {
long beginTime = System.currentTimeMillis();
for (int i = 0; i < dataCount; i++) {
stringRedisTemplate.opsForZSet().add(ZSetKey, "value" + i, i);
}
log.info("使用ZSet类型循环插入{}条数据,总耗时为: {} ms", dataCount, System.currentTimeMillis() - beginTime);
}
使用 opsForZSet().add()
的批量插入方法:
/**
* ZSet测试批量处理插入性能
*
* @param dataCount
*/
public void testBatchInsertOptimizedZSet(int dataCount) {
// 开始计时
long startTime = System.currentTimeMillis();
HashSet<ZSetOperations.TypedTuple<String>> redisBatchData = new HashSet<>();
for (int i = 0; i < dataCount; i++) {
redisBatchData.add(ZSetOperations.TypedTuple.of("value" + i, (double) i));
}
// 一次性批量插入
stringRedisTemplate.opsForZSet().add(ZSetKey, redisBatchData);
log.info("使用ZSet类型批量插入{}条数据,总耗时:{}ms ", dataCount, (System.currentTimeMillis() - startTime));
}
性能对比
插入方式 | 插入数据量 | 耗时(ms) |
---|
循环插入 | 1 万条 | 660+ |
批量插入 | 1 万条 | 50+ |
2.3 Hash 类型
/**
* Hash类型测试循环插入性能
*
* @param dataCount 插入的数据总量
*/
public void testInsertPerformanceHash(int dataCount) {
long beginTime = System.currentTimeMillis();
for (int i = 0; i < dataCount; i++) {
stringRedisTemplate.opsForHash().put(HashKey, "key" + i, "value" + i);
}
log.info("使用Hash类型循环插入{}条数据,总耗时为: {}ms", dataCount, System.currentTimeMillis() - beginTime);
}
/**
* Hash类型测试批量处理插入性能
*
* @param dataCount 插入的数据总量
*/
public void testBatchInsertOptimizedHash(int dataCount) {
long startTime = System.currentTimeMillis();
// 构造批量数据
Map<String, String> hashData = IntStream.range(0, dataCount)
.boxed()
.collect(Collectors.toMap(i -> "key" + i, i -> "value" + i));
// 批量插入
stringRedisTemplate.opsForHash().putAll(HashKey, hashData);
log.info("使用Hash类型批量插入{}条数据,总耗时为: {}ms", dataCount, System.currentTimeMillis() - startTime);
}
性能对比
插入方式 | 插入数据量 | 耗时(ms) |
---|
循环插入 | 1 万条 | 450+ |
批量插入 | 1 万条 | 15+ |
2.4 Set 类型
/**
* Set类型测试循环插入性能
*
* @param dataCount 插入的数据总量
*/
public void testInsertPerformanceSet(int dataCount) {
long beginTime = System.currentTimeMillis();
for (int i = 0; i < dataCount; i++) {
stringRedisTemplate.opsForSet().add("lps::test_set", "value" + i);
}
log.info("使用Set类型循环插入{}条数据,总耗时为: {}ms", dataCount, System.currentTimeMillis() - beginTime);
}
/**
* Set类型测试批量处理插入性能
*
* @param dataCount 插入的数据总量
*/
public void testBatchInsertOptimizedSet(int dataCount) {
long startTime = System.currentTimeMillis();
// 批量插入
stringRedisTemplate.opsForSet().add("lps::test_set", Arrays.toString(IntStream.range(0, dataCount)
.mapToObj(i -> "value" + i).distinct().toArray()));
log.info("使用Set类型批量插入{}条数据,总耗时为: {}ms", dataCount, System.currentTimeMillis() - startTime);
}
性能对比
插入方式 | 插入数据量 | 耗时(ms) |
---|
循环插入 | 1 万条 | 430+ |
批量插入 | 1 万条 | 2+ |
2.5 Geo 类型
/**
* Geo类型测试循环插入性能
*
* @param dataCount 插入的数据总量
*/
public void testInsertPerformanceGeo(int dataCount) {
long beginTime = System.currentTimeMillis();
for (int i = 0; i < dataCount; i++) {
stringRedisTemplate.opsForGeo().add("lps::test_geo", generateValidPoint(i), "location" + i);
}
log.info("使用Geo类型循环插入{}条数据,总耗时为: {}ms", dataCount, System.currentTimeMillis() - beginTime);
}
/**
* 生成合法的 Geo 数据点
*/
private Point generateValidPoint(int index) {
// 生成经度 [-180, 180]
double longitude = (index % 360) - 180;
// 生成纬度 [-85.05112878, 85.05112878]
double latitude = ((index % 170) - 85) * 0.1;
return new Point(longitude, latitude);
}
/**
* Geo类型测试批量处理插入性能
*
* @param dataCount 插入的数据总量
*/
public void testBatchInsertOptimizedGeo(int dataCount) {
long startTime = System.currentTimeMillis();
// 构造批量数据
List<RedisGeoCommands.GeoLocation<String>> geoLocations = IntStream.range(0, dataCount)
.mapToObj(i -> new RedisGeoCommands.GeoLocation<>("location" + i,
generateValidPoint(i)))
.collect(Collectors.toList());
// 批量插入
stringRedisTemplate.opsForGeo().add("lps::test_geo", geoLocations);
log.info("使用Geo类型批量插入{}条数据,总耗时为: {}ms", dataCount, System.currentTimeMillis() - startTime);
}
/**
* 生成合法的 Geo 数据点
*/
private Point generateValidPoint(int index) {
// 生成经度 [-180, 180]
double longitude = (index % 360) - 180;
// 生成纬度 [-85.05112878, 85.05112878]
double latitude = ((index % 170) - 85) * 0.1;
return new Point(longitude, latitude);
}
性能对比
插入方式 | 插入数据量 | 耗时(ms) |
---|
循环插入 | 1 万条 | 496+ |
批量插入 | 1 万条 | 27+ |
2.6 List 类型
/**
* List类型测试循环插入性能
*
* @param dataCount 插入的数据总量
*/
public void testInsertPerformanceList(int dataCount) {
long beginTime = System.currentTimeMillis();
for (int i = 0; i < dataCount; i++) {
stringRedisTemplate.opsForList().rightPush(ListKey, "value" + i);
}
log.info("使用List类型循环插入{}条数据,总耗时为: {}ms", dataCount, System.currentTimeMillis() - beginTime);
}
/**
* List类型测试批量处理插入性能
*
* @param dataCount 插入的数据总量
*/
public void testBatchInsertOptimizedList(int dataCount) {
long startTime = System.currentTimeMillis();
// 构造批量数据
List<String> values = IntStream.range(0, dataCount)
.mapToObj(i -> "value" + i)
.collect(Collectors.toList());
// 批量插入
stringRedisTemplate.opsForList().rightPushAll(ListKey, values);
log.info("使用List类型批量插入{}条数据,总耗时为: {}ms", dataCount, System.currentTimeMillis() - startTime);
}
性能对比
插入方式 | 插入数据量 | 耗时(ms) |
---|
循环插入 | 1 万条 | 429+ |
批量插入 | 1 万条 | 8+ |
3. 执行图片
5.0.14.1版本 - 1w数据插入
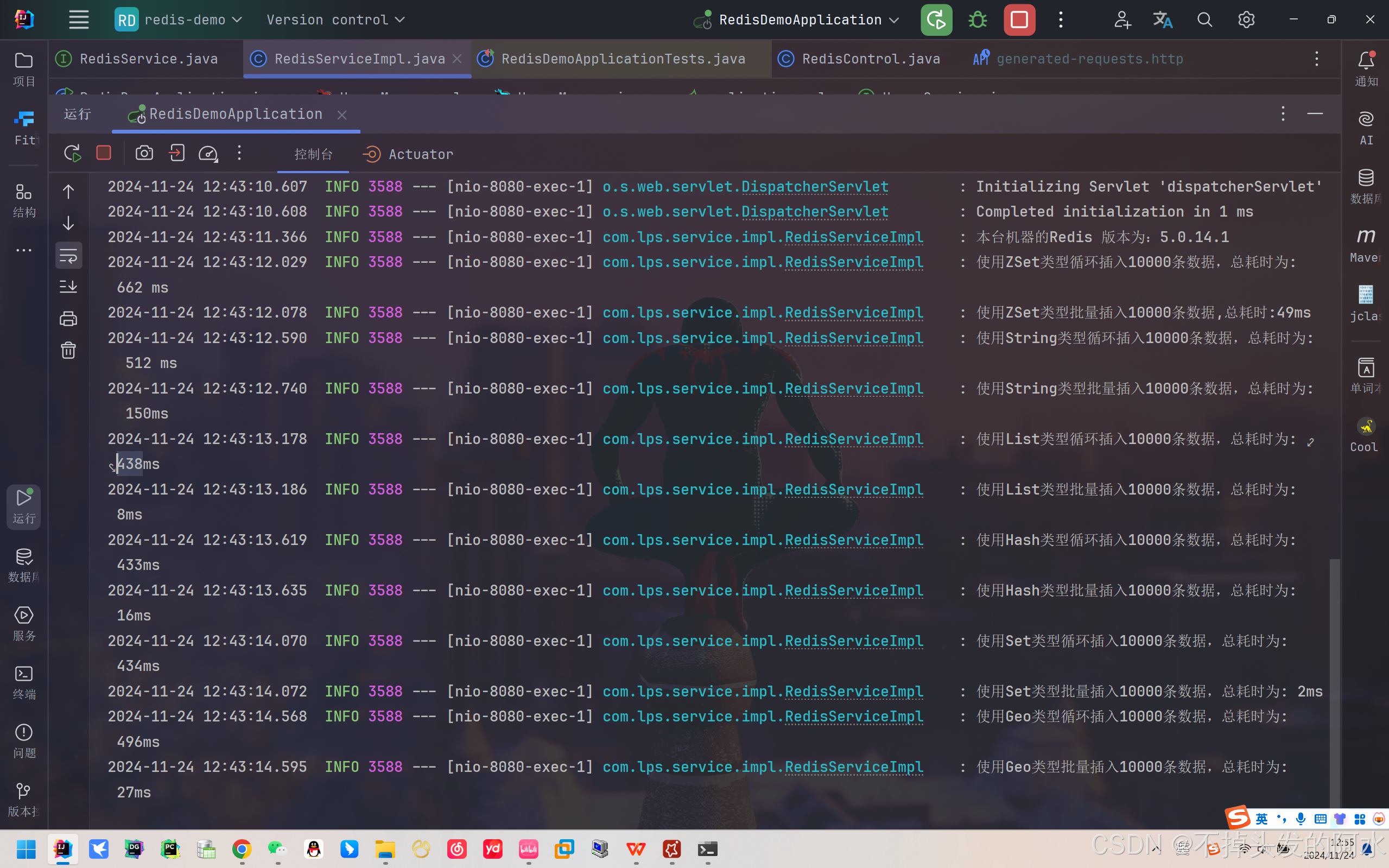
5.0.14.1版本 - 10w数据插入
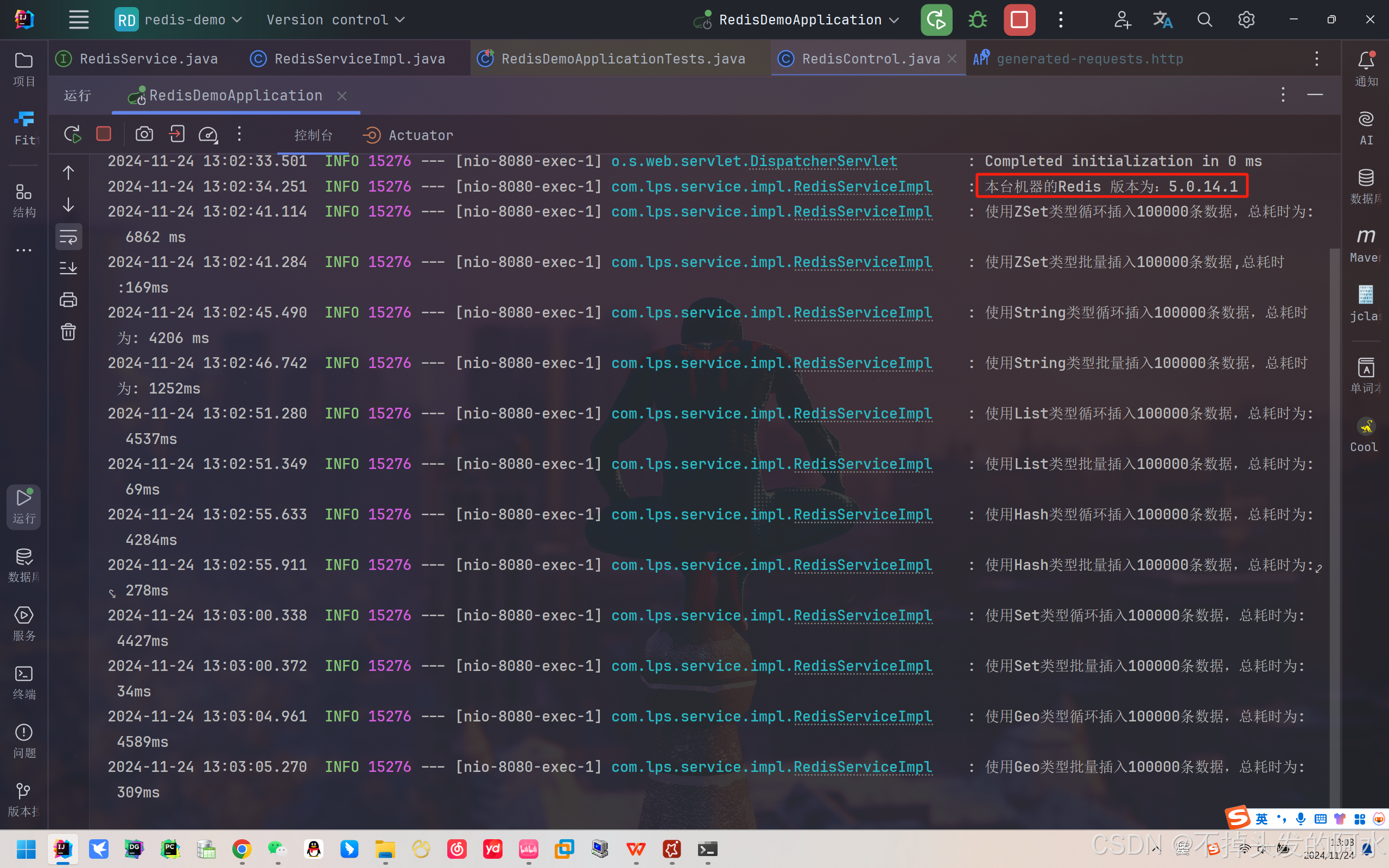
3.2.100版本 - 1w数据插入
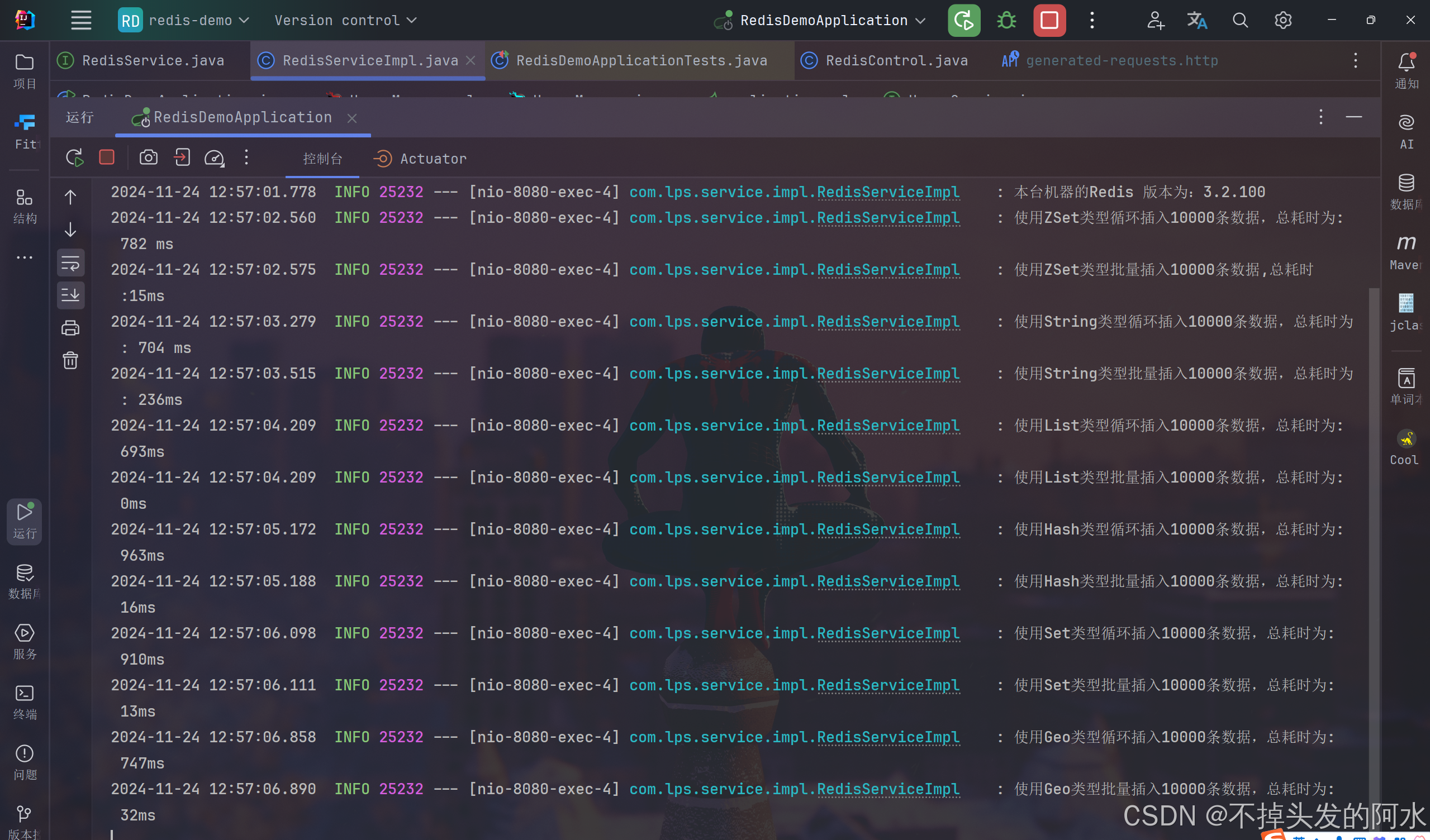
3.2.100版本 - 10w数据插入
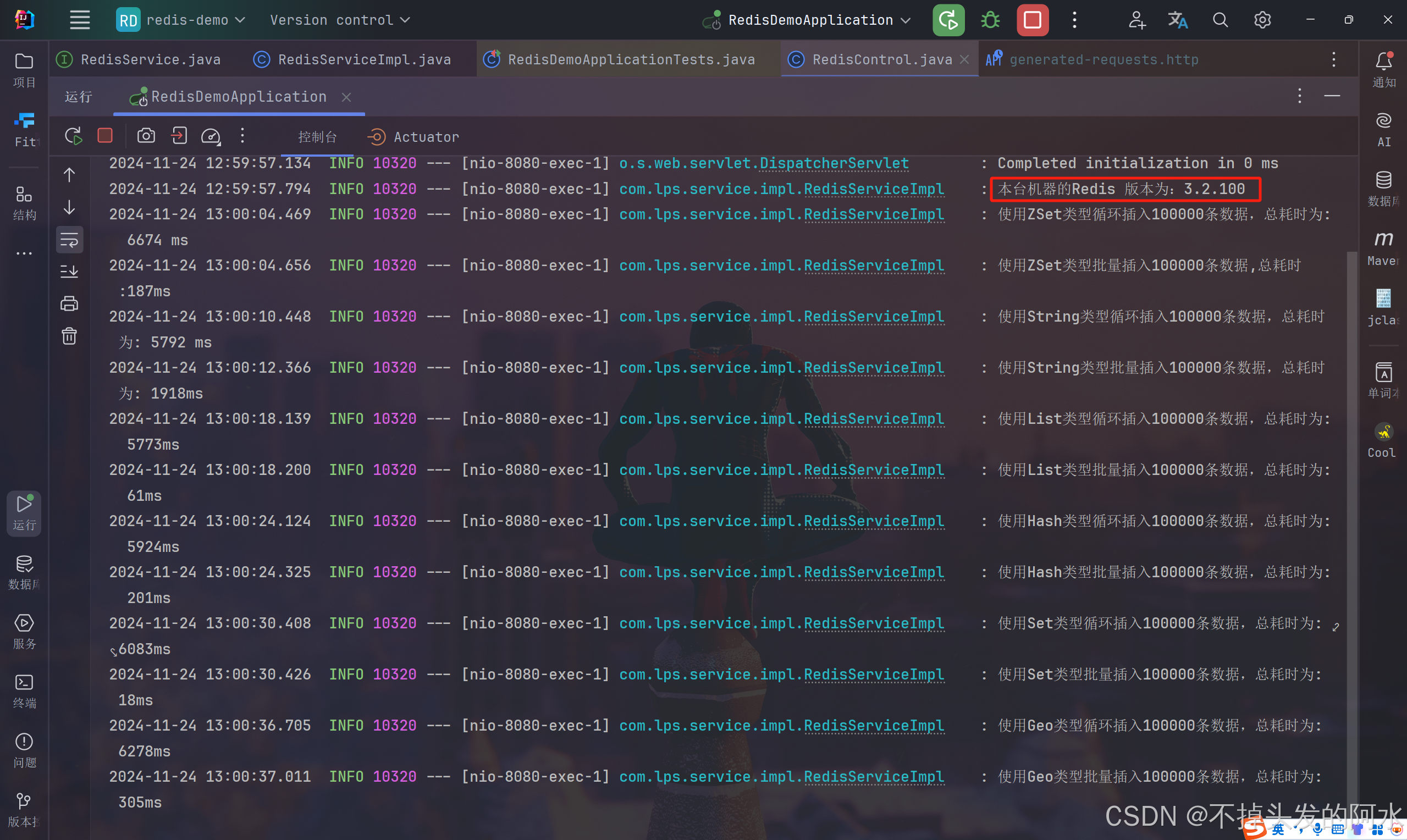
4. 完整类如下
@Service
@Slf4j
public class RedisServiceImpl implements RedisService {
@Resource
private StringRedisTemplate stringRedisTemplate;
public static final String ZSetKey = "lps::test_zset";
public static final String StrKey = "lps::test_str::";
public static final String ListKey = "lps::test_list";
public static final String HashKey = "lps::test_hash";
@Override
public void runInsert(int number) {
checkVersion();
//ZSet类型
testInsertPerformanceZSet(number);
testBatchInsertOptimizedZSet(number);
//String类型
testInsertPerformanceStr(number);
testBatchInsertOptimizedStr(number);
//List类型
testInsertPerformanceList(number);
testBatchInsertOptimizedList(number);
//Hash类型
testInsertPerformanceHash(number);
testBatchInsertOptimizedHash(number);
//Set类型
testInsertPerformanceSet(number);
testBatchInsertOptimizedSet(number);
//Geo类型
testInsertPerformanceGeo(number);
testBatchInsertOptimizedGeo(number);
}
public void checkVersion() {
RedisConnection connection = Objects.requireNonNull(stringRedisTemplate.getConnectionFactory()).getConnection();
String redisVersion = String.valueOf(Objects.requireNonNull(connection.info("server")).get("redis_version"));
log.info("本台机器的Redis 版本为:{}", redisVersion);
}
/**
* ZSet测试循环插入性能
*
* @param dataCount
*/
public void testInsertPerformanceZSet(int dataCount) {
long beginTime = System.currentTimeMillis();
for (int i = 0; i < dataCount; i++) {
stringRedisTemplate.opsForZSet().add(ZSetKey, "value" + i, i);
}
log.info("使用ZSet类型循环插入{}条数据,总耗时为: {} ms", dataCount, System.currentTimeMillis() - beginTime);
}
/**
* ZSet测试批量处理插入性能
*
* @param dataCount
*/
public void testBatchInsertOptimizedZSet(int dataCount) {
// 开始计时
long startTime = System.currentTimeMillis();
HashSet<ZSetOperations.TypedTuple<String>> redisBatchData = new HashSet<>();
for (int i = 0; i < dataCount; i++) {
redisBatchData.add(ZSetOperations.TypedTuple.of("value" + i, (double) i));
}
// 一次性批量插入
stringRedisTemplate.opsForZSet().add(ZSetKey, redisBatchData);
log.info("使用ZSet类型批量插入{}条数据,总耗时:{}ms ", dataCount, (System.currentTimeMillis() - startTime));
}
/**
* String类型测试循环插入性能
*
* @param dataCount
*/
public void testInsertPerformanceStr(int dataCount) {
long beginTime = System.currentTimeMillis();
for (int i = 0; i < dataCount; i++) {
stringRedisTemplate.opsForValue().set(StrKey + i, "value" + i);
}
log.info("使用String类型循环插入{}条数据,总耗时为: {} ms", dataCount, System.currentTimeMillis() - beginTime);
}
/**
* String类型测试批量处理插入性能
*
* @param dataCount 插入的数据总量
*/
public void testBatchInsertOptimizedStr(int dataCount) {
// 开始计时
long startTime = System.currentTimeMillis();
// 使用 Redis Pipeline 进行批量插入
stringRedisTemplate.executePipelined((RedisCallback<Object>) connection -> {
for (int i = 0; i < dataCount; i++) {
// 构造键值对
String key = StrKey + i;
String value = "value" + i;
// 将命令加入 pipeline
connection.stringCommands().set(key.getBytes(), value.getBytes());
}
return null;
});
// 结束计时
long elapsedTime = System.currentTimeMillis() - startTime;
log.info("使用String类型批量插入{}条数据,总耗时为: {}ms", dataCount, elapsedTime);
使用 Lua 脚本删除匹配的键
//String luaScript = "local keys = redis.call('keys', ARGV[1]) " +
// "for i, key in ipairs(keys) do " +
// "redis.call('del', key) " +
// "end " +
// "return #keys";
//DefaultRedisScript<Long> script = new DefaultRedisScript<>(luaScript, Long.class);
//Long deletedKeysCount = redisTemplate.execute(script, Collections.emptyList(), StrKey + "*");
//
//log.info("成功删除前缀为 {} 的所有键,共删除 {} 个键", StrKey, deletedKeysCount);
}
/**
* List类型测试循环插入性能
*
* @param dataCount 插入的数据总量
*/
public void testInsertPerformanceList(int dataCount) {
long beginTime = System.currentTimeMillis();
for (int i = 0; i < dataCount; i++) {
stringRedisTemplate.opsForList().rightPush(ListKey, "value" + i);
}
log.info("使用List类型循环插入{}条数据,总耗时为: {}ms", dataCount, System.currentTimeMillis() - beginTime);
}
/**
* List类型测试批量处理插入性能
*
* @param dataCount 插入的数据总量
*/
public void testBatchInsertOptimizedList(int dataCount) {
long startTime = System.currentTimeMillis();
// 构造批量数据
List<String> values = IntStream.range(0, dataCount)
.mapToObj(i -> "value" + i)
.collect(Collectors.toList());
// 批量插入
stringRedisTemplate.opsForList().rightPushAll(ListKey, values);
log.info("使用List类型批量插入{}条数据,总耗时为: {}ms", dataCount, System.currentTimeMillis() - startTime);
}
/**
* Hash类型测试循环插入性能
*
* @param dataCount 插入的数据总量
*/
public void testInsertPerformanceHash(int dataCount) {
long beginTime = System.currentTimeMillis();
for (int i = 0; i < dataCount; i++) {
stringRedisTemplate.opsForHash().put(HashKey, "key" + i, "value" + i);
}
log.info("使用Hash类型循环插入{}条数据,总耗时为: {}ms", dataCount, System.currentTimeMillis() - beginTime);
}
/**
* Hash类型测试批量处理插入性能
*
* @param dataCount 插入的数据总量
*/
public void testBatchInsertOptimizedHash(int dataCount) {
long startTime = System.currentTimeMillis();
// 构造批量数据
Map<String, String> hashData = IntStream.range(0, dataCount)
.boxed()
.collect(Collectors.toMap(i -> "key" + i, i -> "value" + i));
// 批量插入
stringRedisTemplate.opsForHash().putAll(HashKey, hashData);
log.info("使用Hash类型批量插入{}条数据,总耗时为: {}ms", dataCount, System.currentTimeMillis() - startTime);
}
/**
* Set类型测试循环插入性能
*
* @param dataCount 插入的数据总量
*/
public void testInsertPerformanceSet(int dataCount) {
long beginTime = System.currentTimeMillis();
for (int i = 0; i < dataCount; i++) {
stringRedisTemplate.opsForSet().add("lps::test_set", "value" + i);
}
log.info("使用Set类型循环插入{}条数据,总耗时为: {}ms", dataCount, System.currentTimeMillis() - beginTime);
}
/**
* Set类型测试批量处理插入性能
*
* @param dataCount 插入的数据总量
*/
public void testBatchInsertOptimizedSet(int dataCount) {
long startTime = System.currentTimeMillis();
// 批量插入
stringRedisTemplate.opsForSet().add("lps::test_set", Arrays.toString(IntStream.range(0, dataCount)
.mapToObj(i -> "value" + i).distinct().toArray()));
log.info("使用Set类型批量插入{}条数据,总耗时为: {}ms", dataCount, System.currentTimeMillis() - startTime);
}
/**
* Geo类型测试循环插入性能
*
* @param dataCount 插入的数据总量
*/
public void testInsertPerformanceGeo(int dataCount) {
long beginTime = System.currentTimeMillis();
for (int i = 0; i < dataCount; i++) {
stringRedisTemplate.opsForGeo().add("lps::test_geo", generateValidPoint(i), "location" + i);
}
log.info("使用Geo类型循环插入{}条数据,总耗时为: {}ms", dataCount, System.currentTimeMillis() - beginTime);
}
/**
* Geo类型测试批量处理插入性能
*
* @param dataCount 插入的数据总量
*/
public void testBatchInsertOptimizedGeo(int dataCount) {
long startTime = System.currentTimeMillis();
// 构造批量数据
List<RedisGeoCommands.GeoLocation<String>> geoLocations = IntStream.range(0, dataCount)
.mapToObj(i -> new RedisGeoCommands.GeoLocation<>("location" + i,
generateValidPoint(i)))
.collect(Collectors.toList());
// 批量插入
stringRedisTemplate.opsForGeo().add("lps::test_geo", geoLocations);
log.info("使用Geo类型批量插入{}条数据,总耗时为: {}ms", dataCount, System.currentTimeMillis() - startTime);
}
/**
* 生成合法的 Geo 数据点
*/
private Point generateValidPoint(int index) {
// 生成经度 [-180, 180]
double longitude = (index % 360) - 180;
// 生成纬度 [-85.05112878, 85.05112878]
double latitude = ((index % 170) - 85) * 0.1;
return new Point(longitude, latitude);
}
}
5. 总结与优化建议
- 批量插入性能远远高于循环插入,尤其是在数据量较大时,差距更为明显。
- 使用 Redis Pipeline 或批量操作接口是提高 Redis 性能的有效方法。
- 在批量操作时,尽量将数据准备在内存中再一次性提交到 Redis,减少网络开销。
- Geo 类型插入需注意经纬度合法范围,避免报错。
通过这些优化方法,Redis 的插入性能可以得到显著提升,在高并发场景下尤为重要。