目录
分桶表
分桶表注意事项
hive 分桶表-创建分桶表
hive 排序关键字
hive 排序语句
上机练习
分桶表
分区提供一个隔离数据和优化查询的便利方式。不过,并非所有的数据集都可形
成合理的分区。对于一张表或者分区,Hive 可以进一步组织成桶,也就是更为
细粒度的数据范围划分。
分桶是将数据集分解成更容易管理的若干部分的另一个技术。
分区针对的是数据的存储路径;分桶针对的是数据文件。
分桶表注意事项
分桶策略
Hive 的分桶采用对分桶字段的值进行哈希,然后除以桶的个数求余的方 式决定
该条记录存放在哪个桶当中.
reduce 的个数设置为-1,让 Job 自行决定需要用多少个 reduce 或者将 reduce 的
个数设置为大于等于分桶表的桶数
从 hdfs 中 load 数据到分桶表中,避免本地文件找不到问题
不要使用本地模式
hive 分桶表-创建分桶表
--创建 4 个分桶的分桶表
create table stu_bucket(id int, name string)
clustered by(id)
into 4 buckets
row format delimited fields terminated by '\t';
--设置 mapreduce 数量(二选一)
set mapreduce.job.reduces=3
set mapred.reduce.tasks=3
--向分桶表导入数据
load data inpath
'/student.txt' into table stu_bucket;
hive 排序关键字
hive 排序语句
--使用 order by 排序
select * from student2 order by id
--使用 sort by 排序
select * from student2 sort by class_name desc
--使用 distribute by 分组
set mapreduce.job.reduces=15;
select * from student2 distribute by class_name sort by id desc
insert overwrite
local directory '/root/student2/'
row format delimited fields terminated by '\t'
select * from student2_b
distribute by sex
sort by chinese desc
--使用 cluster by 分组并排序
select * from student2 cluster by class_name
上机练习
然而作业和分桶表并没有关系~
1 清洗函数
2 pyhive 连接函数
3 文件判断
import os,datetime
fpath='/root/'
fname1='2021-12-31.log'
fname2='2021-05-20.log'
fname3='2021-07-02.log'
fname4='2021-07-03.log'
fname5='2021-07-04.log'list_fname=[fname1,fname2,fname3,fname4,fname5]
etl_fname1=f'{fpath}etl_{fname1.strip(".log")}.txt'
etl_fname2=f'{fpath}etl_{fname2.strip(".log")}.txt'
etl_fname3=f'{fpath}etl_{fname3.strip(".log")}.txt'
etl_fname4=f'{fpath}etl_{fname4.strip(".log")}.txt'
etl_fname5=f'{fpath}etl_{fname5.strip(".log")}.txt'
list_etl_fname=[etl_fname1,etl_fname2,etl_fname3,etl_fname4,et
l_fname5]
def etl_data(fpath,fname,etl_fname):
with open(fpath+fname,'r',encoding='utf-8') as f:
with open(etl_fname,'a',encoding='utf-8') as f1:
str1=f.readlines()
for l in str1:
lit=l.split(' ')
# 提取 IP
ip=lit[0]
# 提取时间
date=datetime.datetime.strptime(lit[3],'[%d/%b/
%Y:%H:%M:%S')
date=datetime.datetime.strftime(date,'%Y-%m-%d %
H:%M:%S')
# 提取 URL
url=lit[6]
# 提取系统类型
lit1=l.split('"')
header=lit1[-2]
if header.find('Linux')>=0:
systype='Linux'
elif header.find('Mac OS')>=0:
systype='Mac OS'
elif header.find('Windows')>=0:
systype='Windows'
else:
systype='unknown'
# 提取浏览器类型
if header.find('Chrome')>=0:
browser='Chrome'
elif header.find('Firefox')>=0:
browser='Firefox'
elif header.find('Safari')>=0:
browser='Safari'
elif header.find('Presto')>=0:
browser='Presto'else:
browser='unknown'
# 写入文件
result=f'{ip}\t{date}\t{url}\t{systype}\t{brows
er}\n'
f1.write(result)
f1.close()
f.close()
if __name__=='__main__':
for i in range(5):
etl_data(fpath,list_fname[i],list_etl_fname[i])
load_sql=f"load data local inpath '{list_etl_fname[i]}'
into table log partition
(load_date='{list_fname[i].strip('.log')}')"
hive_command=f'beeline -u
"jdbc:hive2://hadoop100:10000/db_hive" -n root -p 123456 -e
"{load_sql}"'
os.system(hive_command)
4 show partitions log
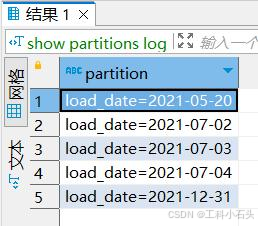
5 select load_date,count(1) from log group by load_date
内存不够跑不了这句,一句一句来吧。分开写查一个都要 50 多秒。
select
count
(
1
)
from
log
where
load_date=
'2021-05-20'
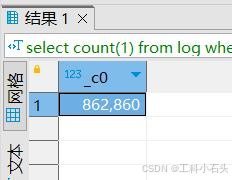
select
count
(
1
)
from
log
where
load_date=
'2021-07-02'
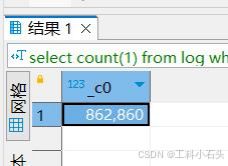
select
count
(
1
)
from
log
where
load_date=
'2021-07-03'
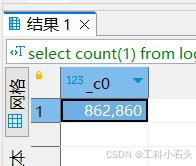
select
count
(
1
)
from
log
where
load_date=
'2021-12-31'
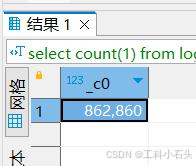
select
count
(
1
)
from
log
where
load_date=
'2021-07-04'
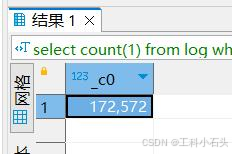