参考链接
ClearML教程:https://blog.csdn.net/qq_40243750/article/details/126445671
b站教学视频:https://www.bilibili.com/video/BV1Mx4y1A7jy/spm_id_from=333.788&vd_source=b52b79abfe565901e6969da2a1191407
开始
github地址:https://github.com/z1069614715/objectdetection_script/tree/master
首先安装timm库:pip install -i https://pypi.tuna.tsinghua.edu.cn/simple timm
可以查看timm中包含的所有库:timm.list_models()
报错1:
解决:直接去这个路径下,删掉__init__.py就可以了,正常来说这是一个空文件,建议删除前检查一下
步骤1:
更改models中的yolov5.py文件
导入timm库
步骤2:
更改models中的yolov5.py文件
先改309行的parse_model函数
def parse_model(d, ch): model_dict, input_channels(3)
# Parse a YOLOv5 model.yaml dictionary
LOGGER.info(f"\n{'':3}{'from':18}{'n':3}{'params':10} {'module':<40}{'arguments':<30}")
anchors, nc, gd, gw, act = d['anchors'], d['nc'], d['depth_multiple'], d['width_multiple'], d.get('activation')
if act:
Conv.default_act = eval(act) redefine default activation, i.e. Conv.default_act = nn.SiLU()
LOGGER.info(f"{colorstr('activation:')} {act}") print
na = (len(anchors[0]) // 2) if isinstance(anchors, list) else anchors number of anchors
no = na * (nc + 5) number of outputs = anchors * (classes + 5)
is_backbone = False
layers, save, c2 = [], [], ch[-1] layers, savelist, ch out
for i, (f, n, m, args) in enumerate(d['backbone'] d['head']): from, number, module, args
try:
t = m
m = eval(m) if isinstance(m, str) else m eval strings
except:
pass
for j, a in enumerate(args):
with contextlib.suppress(NameError):
try:
args[j] = eval(a) if isinstance(a, str) else a eval strings
except:
args[j] = a
n = n_ = max(round(n * gd), 1) if n > 1 else n depth gain
if m in {
Conv, GhostConv, Bottleneck, GhostBottleneck, SPP, SPPF, DWConv, MixConv2d, Focus, CrossConv,
BottleneckCSP, C3, C3TR, C3SPP, C3Ghost, nn.ConvTranspose2d, DWConvTranspose2d, C3x}:
c1, c2 = ch[f], args[0]
if c2 != no: if not output
c2 = make_divisible(c2 * gw, 8)
args = [c1, c2, *args[1:]]
if m in {BottleneckCSP, C3, C3TR, C3Ghost, C3x}:
args.insert(2, n) number of repeats
n = 1
elif m is nn.BatchNorm2d:
args = [ch[f]]
elif m is Concat:
c2 = sum(ch[x] for x in f)
TODO: channel, gw, gd
elif m in {Detect, Segment}:
args.append([ch[x] for x in f])
if isinstance(args[1], int): number of anchors
args[1] = [list(range(args[1] 2))] len(f)
if m is Segment:
args[3] = make_divisible(args[3] gw, 8)
elif m is Contract:
c2 = ch[f] args[0] ** 2
elif m is Expand:
c2 = ch[f] // args[0] ** 2
elif isinstance(m, str):
t = m
m = timm.create_model(m, pretrained=args[0], features_only=True)
c2 = m.feature_info.channels()
elif m in {}:
# m = m(*args)
# c2 = m.channel
else:
c2 = ch[f]
if isinstance(c2, list):
is_backbone = True
m_ = m
m_.backbone = True
else:
m_ = nn.Sequential(*(m(*args) for _ in range(n))) if n > 1 else m(*args) module
t = str(m)[8:-2].replace('__main__.', '') module type
np = sum(x.numel() for x in m_.parameters()) number params
m_.i, m_.f, m_.type, m_.np = i + 4 if is_backbone else i, f, t, np attach index, 'from' index, type, number params
LOGGER.info(f'{i:3}{str(f):18}{n_:3}{np:10.0f} {t:<40}{str(args):<30}') print
save.extend(x % (i + 4 if is_backbone else i) for x in ([f] if isinstance(f, int) else f) if x != -1) append to savelist
layers.append(m_)
if i == 0:
ch = []
if isinstance(c2, list):
ch.extend(c2)
for _ in range(5 len(ch)):
ch.insert(0, 0)
else:
ch.append(c2)
return nn.Sequential(*layers), sorted(save)
步骤3:
更改108行BaseModel类中的_forward_once函数
def _forward_once(self, x, profile=False, visualize=False):
y, dt = [], [] # outputs
for m in self.model:
if m.f != -1: # if not from previous layer
x = y[m.f] if isinstance(m.f, int) else [x if j == -1 else y[j] for j in m.f] # from earlier layers
if profile:
self._profile_one_layer(m, x, dt)
if hasattr(m, 'backbone'):
x = m(x)
for _ in range(5 - len(x)):
x.insert(0, None)
for i_idx, i in enumerate(x):
if i_idx in self.save:
y.append(i)
else:
y.append(None)
x = x[-1]
else:
x = m(x) # run
y.append(x if m.i in self.save else None) # save output
if visualize:
feature_visualization(x, m.type, m.i, save_dir=visualize)
return x
步骤4:
在model文件下创建yovov5-custom.yaml文件
# YOLOv5 🚀 by Ultralytics, GPL-3.0 license
# Parameters
nc: 80 # number of classes
depth_multiple: 0.33 # model depth multiple
width_multiple: 0.25 # layer channel multiple
anchors:
- [10,13, 16,30, 33,23] # P3/8
- [30,61, 62,45, 59,119] # P4/16
- [116,90, 156,198, 373,326] # P5/32
# 0-P1/2
# 1-P2/4
# 2-P3/8
# 3-P4/16
# 4-P5/32
# YOLOv5 v6.0 backbone
backbone:
# [from, number, module, args]
[[-1, 1, resnet34, [False]], # 4
[-1, 1, SPPF, [1024, 5]], # 5
]
# YOLOv5 v6.0 head
head:
[[-1, 1, Conv, [512, 1, 1]], # 6
[-1, 1, nn.Upsample, [None, 2, 'nearest']], # 7
[[-1, 3], 1, Concat, [1]], # cat backbone P4 8
[-1, 3, C3, [512, False]], # 9
[-1, 1, Conv, [256, 1, 1]], # 10
[-1, 1, nn.Upsample, [None, 2, 'nearest']], # 11
[[-1, 2], 1, Concat, [1]], # cat backbone P3 12
[-1, 3, C3, [256, False]], # 13 (P3/8-small)
[-1, 1, Conv, [256, 3, 2]], # 14
[[-1, 10], 1, Concat, [1]], # cat head P4 15
[-1, 3, C3, [512, False]], # 16 (P4/16-medium)
[-1, 1, Conv, [512, 3, 2]], # 17
[[-1, 5], 1, Concat, [1]], # cat head P5 18
[-1, 3, C3, [1024, False]], # 19 (P5/32-large)
[[13, 16, 19], 1, Detect, [nc, anchors]], # Detect(P3, P4, P5)
]
将下面红框中的名称改为你想要的模型。
要确定timm库中有此模型.
a = timm.list_models()
for model in a:
if 'resnet' in model:
print(model)
使用这个函数查看.
步骤5:
更改yolov5.py中的路径
将cfg中的default改为自己的
给peofile和line-profile添加default=True
运行成功: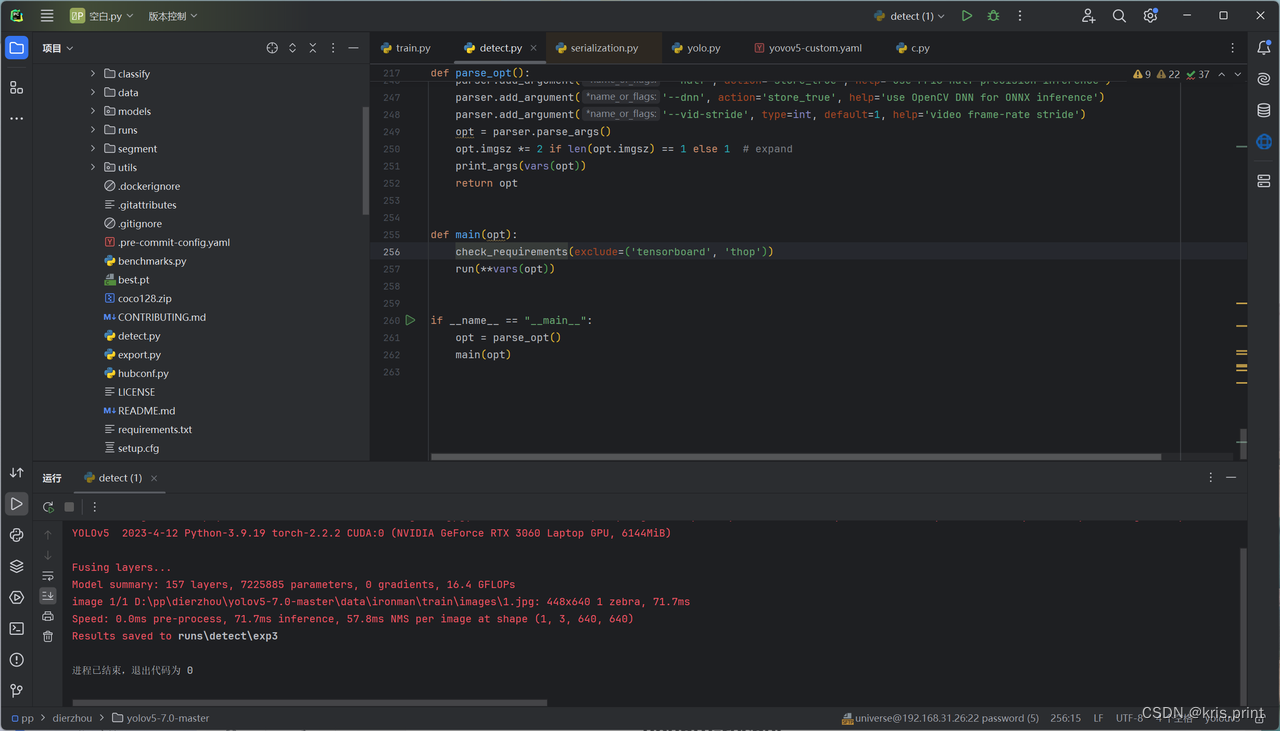
win-resnet
修改loss.data[0]为loss.item()
.view修改为.reshape
修改depth为指定的数,修改epochs提高运行速度
ubuntu-resnet
使用ssh进入conda虚拟环境:conda activate name(环境名称)
ubuntu运行
win-yolov5
报错1:No module named ‘ultralyt///YOLOv8 requirement “ultralytics.utils“ not found, attempting AutoUpdate
解决:ultralytics库未更新,使用pip install --upgrade ultralytics来更新
报错2:UnicodeDecodeError: 'gbk' codec can't decode byte 0x80 in position 234: illegal multibyte sequence
解决:.ym文件中有格式错误的行,删除1-8行
报错3:
NotImplementedError: cannot instantiate 'WindowsPath' on your system
只需要在你执行的python文件开头,加上下面几段代码即可:
import platform
import pathlib
plt = platform.system()
if plt != 'Windows':
pathlib.WindowsPath = pathlib.PosixPath
运行成功
ubuntu-yolov5
conda复制虚拟环境:conda create -n 新环境名称 --clone 旧环境名称
问题1:
解决:
os.environ["GIT_PYTHON_REFRESH"] = "quiet"
问题2: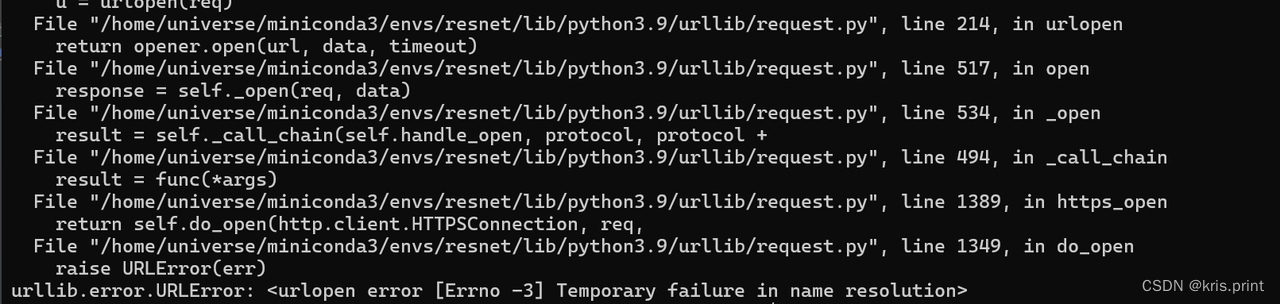
往上找
继续向上翻
这是最开始的报错位置,把这个Arial.ttf下载下来,传到后面的路径里就解决了