01.在翻译、语音识别等将一个时序数据转换为另一个时序数据的任务中,时序数据之间常常存在对应关系
02.Attention 从数据中学习两个时序数据之间的对应关系
03.Attention 使用向量内积(方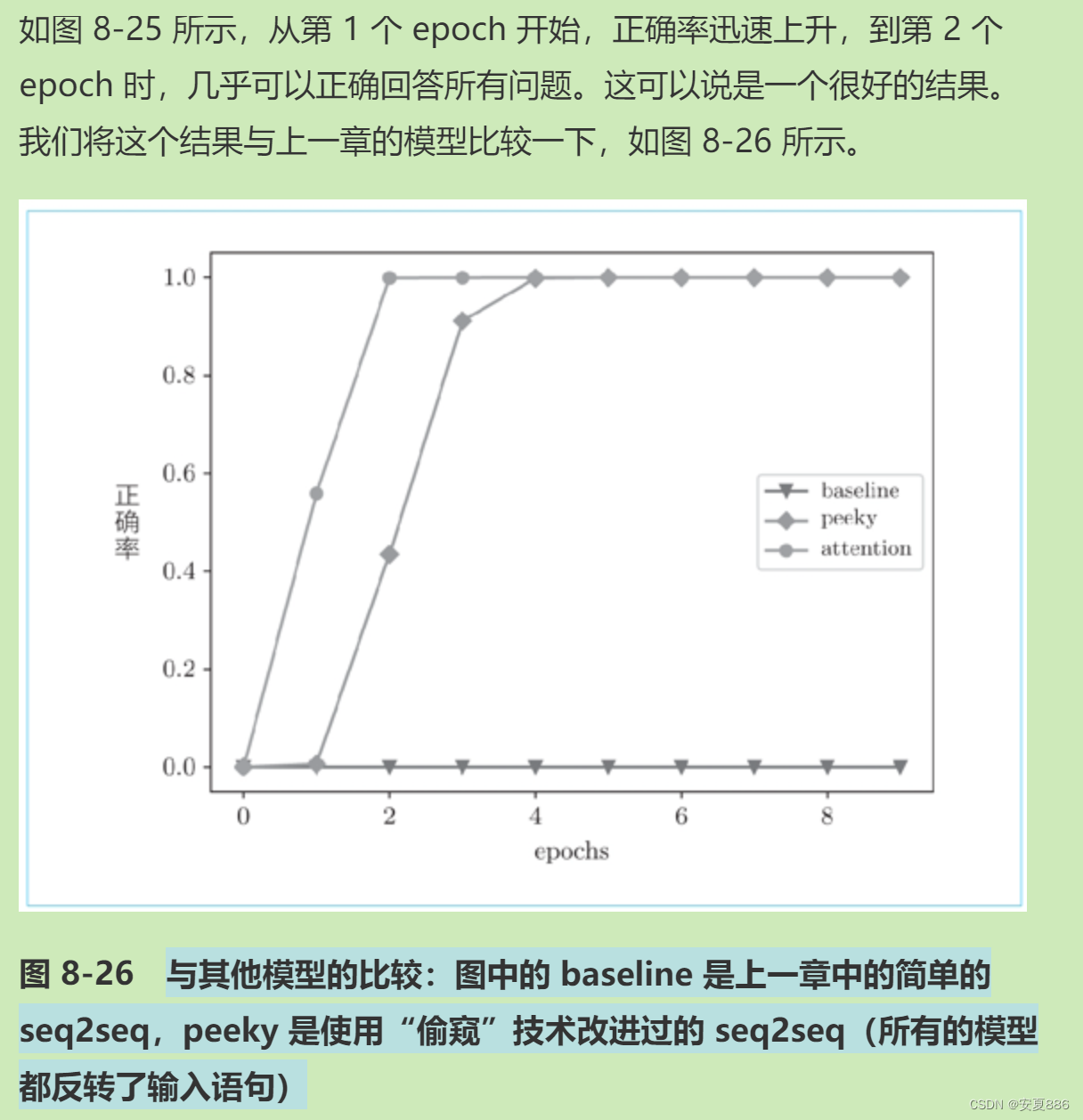
法之一)计算向量之间的相似度,并输出这个相似度的加权和向量
import sys
sys.path.append('..')
from common.layers import Softmax
import numpy as np
N, T, H = 10, 5, 4
hs = np.random.randn(N, T, H)
h = np.random.randn(N, H)
hr = h.reshape(N, 1, H).repeat(T, axis=1)
# hr = h.reshape(N, 1, H) # 广播
t = hs * hr
print(t.shape)
# (10, 5, 4)
s = np.sum(t, axis=2)
print(s.shape)
# (10, 5)
softmax = Softmax()
a = softmax.forward(s)
print(a.shape)
# (10, 5)
class Attention:
def __init__(self):
self.params, self.grads = [], []
self.attention_weight_layer = AttentionWeight()
self.weight_sum_layer = WeightSum()
self.attention_weight = None
def forward(self, hs, h):
a = self.attention_weight_layer.forward(hs, h)
out = self.weight_sum_layer.forward(hs, a)
self.attention_weight = a
return out
def backward(self, dout):
dhs0, da = self.weight_sum_layer.backward(dout)
dhs1, dh = self.attention_weight_layer.backward(da)
dhs = dhs0 + dhs1
return dhs, dh
TimeAttention代码实现:
class TimeAttention:
def __init__(self):
self.params, self.grads = [], []
self.layers = None
self.attention_weights = None
def forward(self, hs_enc, hs_dec):
N, T, H = hs_dec.shape
out = np.empty_like(hs_dec)
self.layers = []
self.attention_weights = []
for t in range(T):
layer = Attention()
out[:, t, :] = layer.forward(hs_enc, hs_dec[:,t,:])
self.layers.append(layer)
self.attention_weights.append(layer.attention_weight)
return out
def backward(self, dout):
N, T, H = dout.shape
dhs_enc = 0
dhs_dec = np.empty_like(dout)
for t in range(T):
layer = self.layers[t]
dhs, dh = layer.backward(dout[:, t, :])
dhs_enc += dhs
dhs_dec[:,t,:] = dh
return dhs_enc, dhs_dec
解释:
04.因为 Attention 中使用的运算是可微分的,所以可以基于误差反向传播法进行学习
05.通过将 Attention 计算出的权重(概率)可视化,可以观察输入与输出之间的对应关系
06.在基于外部存储装置扩展神经网络的研究示例中,Attention 被用来读写内存
小结
本章我们学习了 Attention 的结构,并实现了 Attention 层。然后,我们使用 Attention 实现了 seq2seq,并通过简单的实验,确认了 Attention 的出色效果。另外,我们对模型推理时的 Attention 的权重(概率)进行了可视化。从结果可知,具有 Attention 的模型以与人类相同的方式将注意力放在了必要的信息上。
另外,本章还介绍了有关 Attention 的前沿研究。从多个例子可知,Attention 扩展了深度学习的可能性。Attention 是一种非常有效的技术,具有很大潜力。在深度学习领域,今后 Attention 自己也将吸引更多的“注意力”。
Deep Learning Second Book Finished!