一、前言
sax理论篇:时间序列表征之SAX(Symbolic Aggregate approXimation)算法
二、sax实现
2.1 过程
标准化(将数据转换为高斯分布)
paa
discretization
2.2 标准化
因为原文中采用的breakpoints为
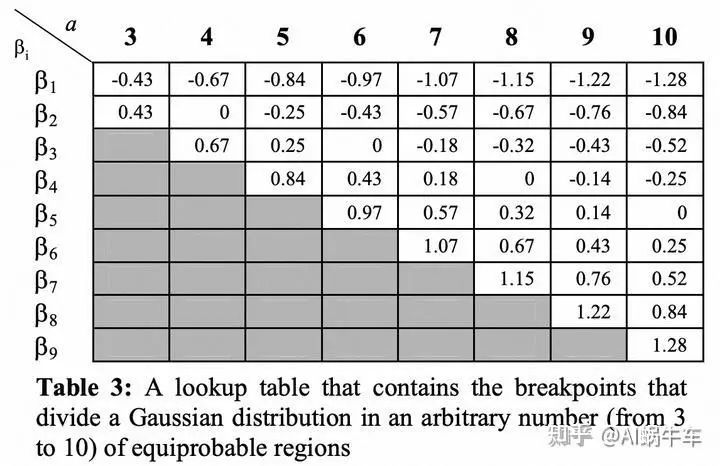
前提假设为:离散化要求产生具有等概率的符号,通过标准化的时间序列具有高斯分布
所以标准的sax之前应该先通过一次标准化得到数据,很多例子这步骤都是直接略过了,因为例子中的数据都是生成的本就具有高斯分布的数据
2.3 paa
这里没啥好讲的,直接看代码
def paa_step_df(self, df):
n = len(df)
start = 0
approximation = []
i = 0
df_array = df.to_numpy()
while start <= n - self.aggregation_sax_size:
thisFrame = np.array(df_array[start:int(start + self.aggregation_sax_size)])
approximation.append(np.mean(thisFrame, axis=0))
i += 1
start = int(i * self.aggregation_sax_size)
df_paa = pd.DataFrame(data=np.array(approximation), columns=df.columns)
return df_paa
2.4 discretization
def discretization_step_df(self, df_paa):
data = {col: [] for col in df_paa.columns}
n = len(df_paa)
for col in df_paa.columns:
dis = []
for t in range(n):
value_for_feature = df_paa[col].iloc[t]
dis.append(self.alphabet[np.searchsorted(self.breakpoints_for_feature,
value_for_feature,
side='left')])
data[col] = dis
df_sax = pd.DataFrame(data=data, index=df_paa.index)
return df_sax
这里有很多可以根据场景调整的点,简单说两种情况
比如数据中极值点有很多,那是否需要先去除噪声,再离散化
如果数据对于标准化有一定的限制,不标准化情况下我们怎么定义breakpoints_for_feature
这些情况可以酌情根据自身场景进行调整,甚至可以在做sax之前对数据做尽可能的观察,比如有几个顶峰,是否存在周期,等采用不一样的sax策略
2.5 sax全部代码
import numpy
from matplotlib import pyplot as plt
from scipy.stats import norm
import pandas as pd
import numpy as np
import math
from sklearn.preprocessing import StandardScaler
class SAX(object):
def __init__(self, alphabet_size=7, aggregation_sax_size=3):
self.alphabet_size = alphabet_size
self.alphabet = np.array([chr(i) for i in range(97, 97 + self.alphabet_size)])
self.aggregation_sax_size = aggregation_sax_size
self.breakpoints_for_feature = norm.ppf(np.linspace(0, 1, alphabet_size + 1)[1:-1])
self.scaler = StandardScaler()
def fit(self, df):
self.scaler.fit(df)
def paa_step_df(self, df):
n = len(df)
start = 0
approximation = []
i = 0
df_array = df.to_numpy()
while start <= n - self.aggregation_sax_size:
thisFrame = np.array(df_array[start:int(start + self.aggregation_sax_size)])
approximation.append(np.mean(thisFrame, axis=0))
i += 1
start = int(i * self.aggregation_sax_size)
df_paa = pd.DataFrame(data=np.array(approximation), columns=df.columns)
return df_paa
def discretization_step_df(self, df_paa):
data = {col: [] for col in df_paa.columns}
n = len(df_paa)
for col in df_paa.columns:
dis = []
for t in range(n):
value_for_feature = df_paa[col].iloc[t]
dis.append(self.alphabet[np.searchsorted(self.breakpoints_for_feature,
value_for_feature,
side='left')])
data[col] = dis
df_sax = pd.DataFrame(data=data, index=df_paa.index)
return df_sax
def process(self, df):
# 1. Normalization
columns = df.columns
df_int = self.scaler.transform(df)
df_int = pd.DataFrame(df_int, columns=columns)
# 2. PAA
df_paa = self.paa_step_df(df_int)
# 3. Discretization
df_sax = self.discretization_step_df(df_paa)
return df_sax
其中alphabet_size为离散的部分个数,aggregation_sax_size为聚合时间点数。
三、 例子
3.1 数据
用两种数据
周期性数据
非周期性趋势数据
# 设置时间序列长度
n_points = 200
# 生成时间索引
time_index = pd.date_range('2023-01-01', periods=n_points, freq='D')
# 生成周期性时间序列数据
# 包含多个正弦波和一些随机噪声
freq1, freq2 = 5, 25 # 频率
amp1, amp2 = 1, 0.5 # 振幅
noise_amp = 0.1 # 噪声振幅
periodic_series = amp1 * np.sin(2 * np.pi * (time_index.dayofyear / 365) * freq1) + \
amp2 * np.sin(2 * np.pi * (time_index.dayofyear / 365) * freq2) + \
noise_amp * np.random.randn(n_points)
# 生成非周期性时间序列数据
# 包括一个向上的趋势和随机噪声
trend_slope = 0.05
non_periodic_series = trend_slope * np.arange(n_points) + \
np.cumsum(noise_amp * np.random.randn(n_points))
# 创建DataFrame
df = pd.DataFrame({
'period': periodic_series,
'no_period': non_periodic_series
}, index=time_index)
# 绘制时间序列
plt.figure(figsize=(14, 6))
plt.subplot(1, 2, 1)
plt.plot(df.index, df['period'])
plt.title('Complex Periodic Time Series')
plt.subplot(1, 2, 2)
plt.plot(df.index, df['no_period'])
plt.title('Complex Non-Periodic Time Series')
plt.tight_layout()
plt.show()
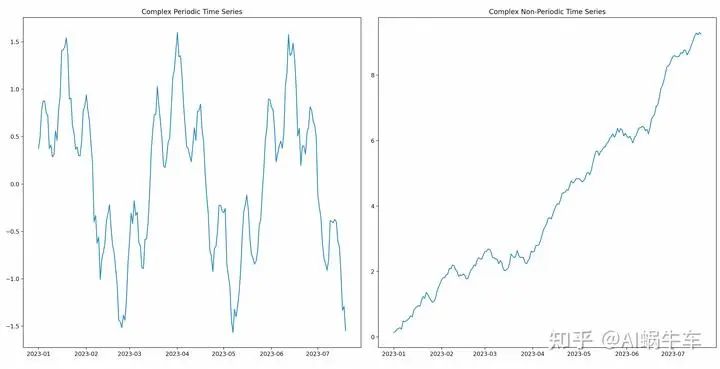
3.2 sax过程
alphabet_size = 12
aggregation_sax_size = 3
sax = SAX(alphabet_size=alphabet_size, aggregation_sax_size=aggregation_sax_size)
sax.fit(df=df)
df_sax = sax.process(df=df)
3.3 可视化
if __name__ == "__main__":
# 设置时间序列长度
n_points = 200
# 生成时间索引
time_index = pd.date_range('2023-01-01', periods=n_points, freq='D')
# 生成周期性时间序列数据
# 包含多个正弦波和一些随机噪声
freq1, freq2 = 5, 25 # 频率
amp1, amp2 = 1, 0.5 # 振幅
noise_amp = 0.1 # 噪声振幅
periodic_series = amp1 * np.sin(2 * np.pi * (time_index.dayofyear / 365) * freq1) + \
amp2 * np.sin(2 * np.pi * (time_index.dayofyear / 365) * freq2) + \
noise_amp * np.random.randn(n_points)
# 生成非周期性时间序列数据
# 包括一个向上的趋势和随机噪声
trend_slope = 0.05
non_periodic_series = trend_slope * np.arange(n_points) + \
np.cumsum(noise_amp * np.random.randn(n_points))
# 创建DataFrame
df = pd.DataFrame({
'period': periodic_series,
'no_period': non_periodic_series
}, index=time_index)
# 绘制时间序列
plt.figure(figsize=(14, 6))
plt.subplot(2, 2, 1)
plt.plot(df.index, df['period'])
plt.title('Complex Periodic Time Series')
plt.subplot(2, 2, 2)
plt.plot(df.index, df['no_period'])
plt.title('Complex Non-Periodic Time Series')
for index, fea in enumerate(['period', 'no_period']):
alphabet_size = 12
aggregation_sax_size = 3
sax = SAX(alphabet_size=alphabet_size, aggregation_sax_size=aggregation_sax_size)
sax.fit(df=df)
df_sax = sax.process(df=df)
plt.subplot(2, 2, 2 + index + 1)
alphabet = np.array([chr(i) for i in range(97, 97 + alphabet_size)])
# Assign an integer to each letter (for plotting purposes)
d = dict(zip([np.where(alphabet == e)[0][0]
for e in alphabet], alphabet))
reverse_d = {v: k for k, v in d.items()}
sax_reverse = [reverse_d[e] for e in df_sax[fea].to_list()]
plt.step(np.arange(len(df_sax)), sax_reverse, where='post',
c='k', linewidth=2, alpha=0.8)
plt.xticks(rotation='vertical')
plt.yticks(range(len(alphabet)), alphabet)
plt.grid()
plt.tight_layout()
plt.show()
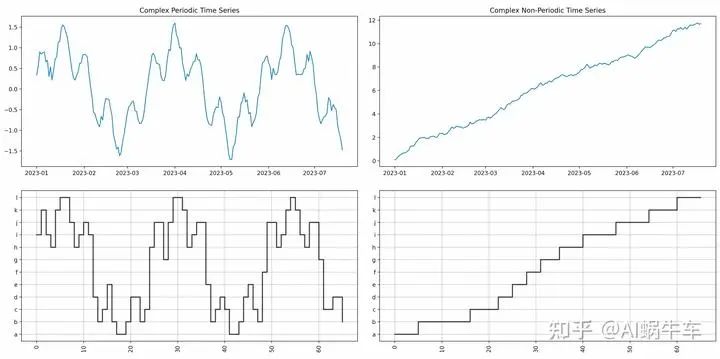
3.4 全部代码
import numpy
from matplotlib import pyplot as plt
from scipy.stats import norm
import pandas as pd
import numpy as np
import math
from sklearn.preprocessing import StandardScaler
class SAX(object):
def __init__(self, alphabet_size=7, aggregation_sax_size=3):
self.alphabet_size = alphabet_size
self.alphabet = np.array([chr(i) for i in range(97, 97 + self.alphabet_size)])
self.aggregation_sax_size = aggregation_sax_size
self.breakpoints_for_feature = norm.ppf(np.linspace(0, 1, alphabet_size + 1)[1:-1])
self.scaler = StandardScaler()
def fit(self, df):
self.scaler.fit(df)
def paa_step_df(self, df):
n = len(df)
start = 0
approximation = []
i = 0
df_array = df.to_numpy()
while start <= n - self.aggregation_sax_size:
thisFrame = np.array(df_array[start:int(start + self.aggregation_sax_size)])
approximation.append(np.mean(thisFrame, axis=0))
i += 1
start = int(i * self.aggregation_sax_size)
df_paa = pd.DataFrame(data=np.array(approximation), columns=df.columns)
return df_paa
def discretization_step_df(self, df_paa):
data = {col: [] for col in df_paa.columns}
n = len(df_paa)
for col in df_paa.columns:
dis = []
for t in range(n):
value_for_feature = df_paa[col].iloc[t]
dis.append(self.alphabet[np.searchsorted(self.breakpoints_for_feature,
value_for_feature,
side='left')])
data[col] = dis
df_sax = pd.DataFrame(data=data, index=df_paa.index)
return df_sax
def process(self, df):
# 1. Normalization
columns = df.columns
df_int = self.scaler.transform(df)
df_int = pd.DataFrame(df_int, columns=columns)
# 2. PAA
df_paa = self.paa_step_df(df_int)
# 3. Discretization
df_sax = self.discretization_step_df(df_paa)
return df_sax
if __name__ == "__main__":
# 设置时间序列长度
n_points = 200
# 生成时间索引
time_index = pd.date_range('2023-01-01', periods=n_points, freq='D')
# 生成周期性时间序列数据
# 包含多个正弦波和一些随机噪声
freq1, freq2 = 5, 25 # 频率
amp1, amp2 = 1, 0.5 # 振幅
noise_amp = 0.1 # 噪声振幅
periodic_series = amp1 * np.sin(2 * np.pi * (time_index.dayofyear / 365) * freq1) + \
amp2 * np.sin(2 * np.pi * (time_index.dayofyear / 365) * freq2) + \
noise_amp * np.random.randn(n_points)
# 生成非周期性时间序列数据
# 包括一个向上的趋势和随机噪声
trend_slope = 0.05
non_periodic_series = trend_slope * np.arange(n_points) + \
np.cumsum(noise_amp * np.random.randn(n_points))
# 创建DataFrame
df = pd.DataFrame({
'period': periodic_series,
'no_period': non_periodic_series
}, index=time_index)
# 绘制时间序列
plt.figure(figsize=(14, 6))
plt.subplot(2, 2, 1)
plt.plot(df.index, df['period'])
plt.title('Complex Periodic Time Series')
plt.subplot(2, 2, 2)
plt.plot(df.index, df['no_period'])
plt.title('Complex Non-Periodic Time Series')
for index, fea in enumerate(['period', 'no_period']):
alphabet_size = 12
aggregation_sax_size = 3
sax = SAX(alphabet_size=alphabet_size, aggregation_sax_size=aggregation_sax_size)
sax.fit(df=df)
df_sax = sax.process(df=df)
plt.subplot(2, 2, 2 + index + 1)
alphabet = np.array([chr(i) for i in range(97, 97 + alphabet_size)])
# Assign an integer to each letter (for plotting purposes)
d = dict(zip([np.where(alphabet == e)[0][0]
for e in alphabet], alphabet))
reverse_d = {v: k for k, v in d.items()}
sax_reverse = [reverse_d[e] for e in df_sax[fea].to_list()]
plt.step(np.arange(len(df_sax)), sax_reverse, where='post',
c='k', linewidth=2, alpha=0.8)
plt.xticks(rotation='vertical')
plt.yticks(range(len(alphabet)), alphabet)
plt.grid()
plt.tight_layout()
plt.show()
推荐阅读:
我的2022届互联网校招分享
我的2021总结
浅谈算法岗和开发岗的区别
互联网校招研发薪资汇总
公众号:AI蜗牛车
保持谦逊、保持自律、保持进步
发送【蜗牛】获取一份《手把手AI项目》(AI蜗牛车著)
发送【1222】获取一份不错的leetcode刷题笔记
发送【AI四大名著】获取四本经典AI电子书