pl_vio线特征·part II
- 0.引言
- 4.线段残差对位姿的导数
- 4.1.直线的观测模型和误差
- 4.2.误差雅克比推导
- 4.3.误差雅可比求导简洁版(不含imu坐标系转换)
- 4.4.相关代码
0.引言
- pl_vio线特征·part I
现在CSDN有字数限制了,被迫拆分为两篇文章。
4.线段残差对位姿的导数
- 这一小节理论部分来自这里,当然也是来自原论文。
4.1.直线的观测模型和误差
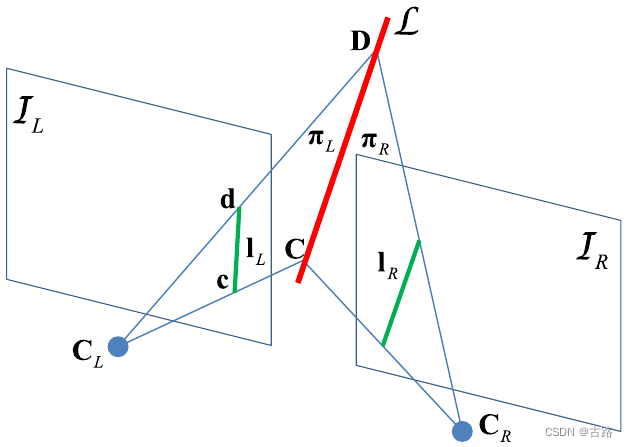
图2 空间直线投影到像素平面
要想知道线特征的观测模型,我们需要知道线特征从归一化平面到像素平面的投影内参矩阵
K
\cal{K}
K 。如图2,点
C
C
C 和
D
D
D 是直线
L
=
(
n
⊤
,
d
⊤
)
⊤
\mathcal{L} =(\mathbf{n}^{\top},\mathbf{d}^{\top})^{\top}
L=(n⊤,d⊤)⊤ 上两点,点
c
c
c 和
d
d
d 是它们在像素平面上的投影。
c
=
K
C
c = KC
c=KC,
d
=
K
D
d=KD
d=KD ,
K
K
K是相机的内参矩阵。
n
=
[
C
]
×
D
,
l
=
[
l
1
l
2
l
3
]
=
[
c
]
×
d
\mathbf{n}=[C]_{\times}D ,\mathscr{l} = \left[\begin{matrix}l_1&l_2&l_3\end{matrix}\right]=[c]_{\times}d
n=[C]×D,l=[l1l2l3]=[c]×d 。那么有
l = K n = [ f y 0 0 0 f x 0 − f y c x − f x c y f x f y ] n \mathscr{l} = \mathcal{K} \mathbf{n} =\left[ \begin{array}{ccc}{f_{y}} & {0} & {0} \\ {0} & {f_{x}} & {0} \\ {-f_{y} c_{x}} & {-f_{x} c_{y}} & {f_{x} f_{y}}\end{array}\right] \mathbf{n} l=Kn= fy0−fycx0fx−fxcy00fxfy n
l i = c i × d i = ( K C n ) × ( K D n ) = [ f x X C + c x f y Y C + c y 1 ] × [ f x X D + c x f y Y D + c y 1 ] = [ 0 − 1 ( f y Y C + c y ) 1 0 − ( f x X C + c x ) − ( f y Y C + c y ) ( f x X C + c x ) 0 ] [ f x X D + c x f y Y D + c y 1 ] = [ f y ( Y C − Y D ) f x ( X C − X D ) f x f y ( X C Y D − Y C X D ) + f x c y ( X D − X C ) + f y c x ( Y D − Y C ) ] = [ f y 0 0 0 f x 0 − f y c x − f x c y f x f y ] [ Y D − Y C X D − X C X C Y D − Y C X D ] = [ f y 0 0 0 f x 0 − f y c x − f x c y f x f y ] [ X C Y C 1 ] × [ X D Y D 1 ] = K ( C n × D n ) = K n \begin{aligned}{l}^i = c{^i} \times d{^i} &= (KC{^n}) \times (KD{^n}) \\ &=\begin{bmatrix}fxX_C+cx \\ fyY_C+cy \\ 1\end{bmatrix}_{\times}\begin{bmatrix}fxX_D+cx \\ fyY_D+cy \\ 1\end{bmatrix} \\ &=\begin{bmatrix}0 & -1 & (fyY_C+cy) \\ 1 & 0 & -(fxX_C+cx) \\ -(fyY_C+cy) & (fxX_C+cx) & 0 \end{bmatrix}\begin{bmatrix}fxX_D+cx \\ fyY_D+cy \\ 1\end{bmatrix} \\ &=\begin{bmatrix}fy(Y_C-Y_D) \\ fx(X_C-X_D) \\ fxfy(X_CY_D-Y_CX_D)+fxcy(X_D-X_C)+fycx(Y_D-Y_C) \end{bmatrix} \\ &=\begin{bmatrix}fy & 0 & 0 \\ 0 & fx & 0 \\ -fycx & -fxcy & fxfy \end{bmatrix}\begin{bmatrix}Y_D-Y_C \\ X_D-X_C \\ X_CY_D-Y_CX_D \end{bmatrix} \\ &=\begin{bmatrix}fy & 0 & 0 \\ 0 & fx & 0 \\ -fycx & -fxcy & fxfy \end{bmatrix} \begin{bmatrix}X_C \\ Y_C \\ 1 \end{bmatrix}_{\times} \begin{bmatrix}X_D \\ Y_D \\ 1 \end{bmatrix} \\ &=\mathcal{K}(C{^n} \times D{^n}) \\ &=\mathcal{K} \mathbf{n} \end{aligned} li=ci×di=(KCn)×(KDn)= fxXC+cxfyYC+cy1 × fxXD+cxfyYD+cy1 = 01−(fyYC+cy)−10(fxXC+cx)(fyYC+cy)−(fxXC+cx)0 fxXD+cxfyYD+cy1 = fy(YC−YD)fx(XC−XD)fxfy(XCYD−YCXD)+fxcy(XD−XC)+fycx(YD−YC) = fy0−fycx0fx−fxcy00fxfy YD−YCXD−XCXCYD−YCXD = fy0−fycx0fx−fxcy00fxfy XCYC1 × XDYD1 =K(Cn×Dn)=Kn
上式表明,直线的线投影只和法向量有关和方向向量无关。
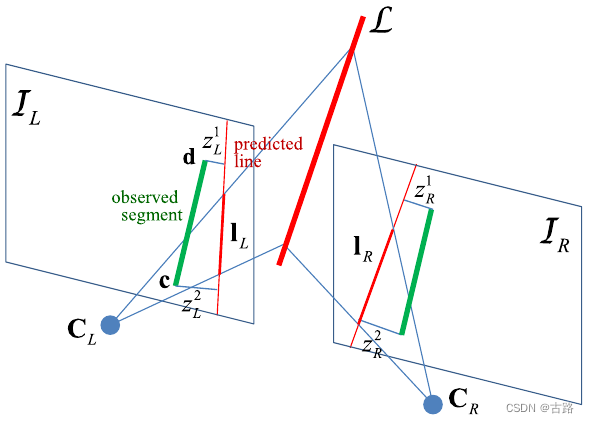
关于投影的误差,我们不可以直接从两幅图像的线段中得到,因为同一条直线在不同图像线段的长度和大小都是不一样的。衡量线的投影误差必须从空间中重投影回当前的图像中才能定义误差。在给定世界坐标系下的空间直线 L l w \mathcal{L}^w_l Llw 和正交表示 O l \mathcal{O}_l Ol ,我们首先使用外参(这也是我们需要优化求解的东西) T c w = [ R c w p c w 0 1 ] T_{cw} = \left[\begin{matrix}R_{cw} & p_{cw}\\0&1 \end{matrix}\right] Tcw=[Rcw0pcw1] 将直线变换到相机归一化平面下的观测 c i c_i ci 坐标下。然后再将直线利用相机内参投影到成像平面上得到投影线段 l l c i \mathscr{l}_l^{c_i} llci ,然后我们就得到了线的投影误差。我们将线的投影误差定义为图像中观测线段的端点到从空间重投影回像素平面的预测直线的距离。
r l ( z L l c i , X ) = [ d ( s l c i , l l c i ) d ( e l c i , l l c i ) ] d ( s , 1 ) = s ⊤ l l 1 2 + l 2 2 \mathbf{r}_{l}\left(\mathbf{z}_{\mathcal{L}_{l}}^{c_{i}}, \mathcal{X}\right)=\left[ \begin{array}{l}{d\left(\mathbf{s}_{l}^{c_{i}}, \mathbf{l}_{l}^{c_{i}}\right)} \\ {d\left(\mathbf{e}_{l}^{c_{i}}, \mathbf{l}_{l}^{c_{i}}\right)}\end{array}\right]\\d(\mathbf{s}, 1)=\frac{\mathbf{s}^{\top} \mathbf{l}}{\sqrt{l_{1}^{2}+l_{2}^{2}}} rl(zLlci,X)=[d(slci,llci)d(elci,llci)]d(s,1)=l12+l22s⊤l
其中 s l c i \mathbf{s}_l^{c_i} slci 和 e l c i \mathbf{e}_l^{c_i} elci 是图像中观测到的线段端点, l l c i \mathbf{l}_l^{c_i} llci 是重投影的预测的直线。
double FeatureManager::reprojection_error( Vector4d obs, Matrix3d Rwc, Vector3d twc, Vector6d line_w ) {
double error = 0;
Vector3d n_w, d_w;
n_w = line_w.head(3);
d_w = line_w.tail(3);
Vector3d p1, p2;
p1 << obs[0], obs[1], 1;
p2 << obs[2], obs[3], 1;
// 根据外参将line从世界坐标系转到相机归一化平面坐标系
Vector6d line_c = plk_from_pose(line_w,Rwc,twc);
Vector3d nc = line_c.head(3);
double sql = nc.head(2).norm();
nc /= sql;
error += fabs( nc.dot(p1) );
error += fabs( nc.dot(p2) );
return error / 2.0;
}
这里误差是归一化平面坐标系的误差,因此观测也应该要求是归一化平面,注意中间有个从像素坐标系到归一化平面坐标系的转换,这里没列出来。
误差求解函数在这里。
这个函数实际上只用在了外点剔除这里,真正的优化误差求解是在优化器那里定义的。而且感觉这里的实现坐标有点问题?
4.2.误差雅克比推导
如果要优化的话,需要知道误差的雅克比矩阵:
线特征在VIO下根据链式求导法则:
J l = ∂ r l ∂ l c i ∂ l c i ∂ L c i [ ∂ L c i ∂ δ x i ∂ L c i ∂ L w ∂ L w ∂ δ O ] \mathbf{J}_{l}=\frac{\partial \mathbf{r}_{l}}{\partial \mathbf{l}^{c_{i}}} \frac{\partial \mathbf{l}^{c_{i}}}{\partial \mathcal{L}^{c_{i}}}\left[\frac{\partial \mathcal{L}^{c_{i}}}{\partial \delta \mathbf{x}^{i}} \quad \frac{\partial \mathcal{L}^{c_{i}}}{\partial \mathcal{L}^{w}} \frac{\partial \mathcal{L}^{w}}{\partial \delta \mathcal{O}}\right] Jl=∂lci∂rl∂Lci∂lci[∂δxi∂Lci∂Lw∂Lci∂δO∂Lw]
其中第一项 ∂ r l ∂ l c i \frac{\partial \mathbf{r}_{l}}{\partial \mathbf{l}^{c_{i}}} ∂lci∂rl ,因为
r l = [ s T l l 1 2 + l 2 2 e T l l 1 2 + l 2 2 ] = [ u s l 1 + v s l 2 l 1 2 + l 2 2 u e l 1 + v e l 2 l 1 2 + l 2 2 ] s = [ u s v s 1 ] e = [ u e v e 1 ] l = [ l 1 l 2 l 3 ] \mathbf{r}_l = \left[ \begin{matrix} \frac{\mathbf{s}^T\mathbf{l} }{\sqrt{l_1^2+l_2^2}} \\ \frac{\mathbf{e}^T\mathbf{l} }{\sqrt{l_1^2+l_2^2}} \end{matrix} \right] = \left[ \begin{matrix} \frac{u_sl_1+v_sl_2 }{\sqrt{l_1^2+l_2^2}} \\ \frac{u_el_1+v_el_2 }{\sqrt{l_1^2+l_2^2}} \end{matrix} \right] \\ \mathbf{s} = \left[\begin{matrix} u_s&v_s&1 \end{matrix} \right] \\ \mathbf{e} = \left[\begin{matrix} u_e&v_e&1 \end{matrix} \right] \\ \mathbf{l} = \left[\begin{matrix} l_1&l_2&l_3 \end{matrix} \right] rl= l12+l22sTll12+l22eTl = l12+l22usl1+vsl2l12+l22uel1+vel2 s=[usvs1]e=[ueve1]l=[l1l2l3]
所以:
∂ r l ∂ l = [ ∂ r 1 ∂ l 1 ∂ r 1 ∂ l 2 ∂ r 1 ∂ l 3 ∂ r 2 ∂ l 1 ∂ r 2 ∂ l 2 ∂ r 2 ∂ l 3 ] = [ − l 1 s l ⊤ l ( l 1 2 + l 2 2 ) ( 3 2 ) + u s ( l 1 2 + l 2 2 ) ( 1 2 ) − l 2 s l ⊤ l ( l 1 2 + l 2 2 ) ( 3 2 ) + v s ( l 1 2 + l 2 2 ) ( 1 2 ) 1 ( l 1 2 + l 2 2 ) ( 1 2 ) − l 1 e l ⊤ l ( l 1 2 + l 2 2 ) ( 3 2 ) + e s ( l 1 2 + l 2 2 ) ( 1 2 ) − l 2 e l ⊤ l ( l 1 2 + l 2 2 ) ( 3 2 ) + v e ( l 1 2 + l 2 2 ) ( 1 2 ) 1 ( l 1 2 + l 2 2 ) ( 1 2 ) ] 2 × 3 \begin{align} \frac{\partial \mathbf{r}_{l}}{\partial \mathbf{l}} &=\left[ \begin{array}{lll}{\frac{\partial r_{1}}{\partial l_{1}}} & {\frac{\partial r_{1}}{\partial l_{2}}} & {\frac{\partial r_{1}}{\partial l_{3}}} \\ {\frac{\partial r_{2}}{\partial l_{1}}} & {\frac{\partial r_{2}}{\partial l_{2}}} & {\frac{\partial r_{2}}{\partial l_{3}}}\end{array}\right] \\&=\left[\begin{matrix} \frac{-l_{1} \mathbf{s}_{l}^{\top} \mathbf{l}}{\left(l_{1}^{2}+l_{2}^{2}\right)^{\left(\frac{3}{2}\right)}}+\frac{u_{s}}{\left(l_{1}^{2}+l_{2}^{2}\right)^{\left(\frac{1}{2}\right)}} & \frac{-l_{2} \mathbf{s}_{l}^{\top} \mathbf{l}}{\left(l_{1}^{2}+l_{2}^{2}\right)^{\left(\frac{3}{2}\right)}}+\frac{v_{s}}{\left(l_{1}^{2}+l_{2}^{2}\right)^{\left(\frac{1}{2}\right)}} & \frac{1}{\left(l_{1}^{2}+l_{2}^{2}\right)^{\left(\frac{1}{2}\right)}} \\ \frac{-l_{1} \mathbf{e}_{l}^{\top} \mathbf{l}}{\left(l_{1}^{2}+l_{2}^{2}\right)^{\left(\frac{3}{2}\right)}}+\frac{e_{s}}{\left(l_{1}^{2}+l_{2}^{2}\right)^{\left(\frac{1}{2}\right)}} & \frac{-l_{2} \mathbf{e}_{l}^{\top} \mathbf{l}}{\left(l_{1}^{2}+l_{2}^{2}\right)^{\left(\frac{3}{2}\right)}}+\frac{v_{e}}{\left(l_{1}^{2}+l_{2}^{2}\right)^{\left(\frac{1}{2}\right)}} & \frac{1}{\left(l_{1}^{2}+l_{2}^{2}\right)^{\left(\frac{1}{2}\right)}} \end{matrix}\right]_{2\times3} \end{align} ∂l∂rl=[∂l1∂r1∂l1∂r2∂l2∂r1∂l2∂r2∂l3∂r1∂l3∂r2]= (l12+l22)(23)−l1sl⊤l+(l12+l22)(21)us(l12+l22)(23)−l1el⊤l+(l12+l22)(21)es(l12+l22)(23)−l2sl⊤l+(l12+l22)(21)vs(l12+l22)(23)−l2el⊤l+(l12+l22)(21)ve(l12+l22)(21)1(l12+l22)(21)1 2×3
第二项 ∂ l c i ∂ L c i \frac{\partial \mathbf{l}^{c_{i}}}{\partial \mathcal{L}^{c_{i}}} ∂Lci∂lci(像素坐标到相机归一化坐标,相差一个映射矩阵) ,因为
l = K n L = [ n d ] \mathbf{l} = \mathcal{K}\mathbf{n} \\ \mathcal{L} = \left[\begin{matrix} \mathbf{n} & \mathbf{d}\end{matrix}\right] l=KnL=[nd]
所以:
∂ l c i ∂ L i c i = [ ∂ l n ∂ l d ] = [ K 0 ] 3 × 6 \begin{align} \frac{\partial \mathrm{l}^{c_{i}}}{\partial \mathcal{L}_{i}^{c_{i}}}&=\left[ \begin{matrix} \frac{\partial \mathbf{l}}{\mathbf{n}} &\frac{\partial \mathbf{l}}{\mathbf{d}} \end{matrix} \right] \\&=\left[ \begin{array}{ll}{\mathcal{K}} & {0}\end{array}\right]_{3 \times 6} \end{align} ∂Lici∂lci=[n∂ld∂l]=[K0]3×6
最后一项矩阵包含两个部分,一个是相机坐标系下线特征对的旋转和平移的误差导数,第二个是直线对正交表示的四个参数增量的导数
第一部分中,
δ x i = [ δ p , δ θ , δ v , δ b a b i , δ b g b i ] \delta \mathbf{x}_{i}=\left[\delta \mathbf{p}, \delta \boldsymbol{\theta}, \delta \mathbf{v}, \delta \mathbf{b}_{a}^{b_{i}}, \delta \mathbf{b}_{g}^{b_{i}}\right] δxi=[δp,δθ,δv,δbabi,δbgbi]
在VIO中,如果要计算线特征的重投影误差,需要将在世界坐标系 w w w 下的线特征变换到IMU坐标系 b b b 下,再用外参数 T b c \bf{T}_{bc} Tbc 变换到相机坐标系 c c c 下。所以
L c = T b c − 1 T w b − 1 L w = T b c − 1 [ R w b ⊤ ( n w + [ d w ] × p w b ) R w b ⊤ d w ] 6 × 1 \begin{aligned} \mathcal{L}_{c} &=\mathcal{T}_{b c}^{-1} \mathcal{T}_{w b}^{-1} \mathcal{L}_{w} \\ &=\mathcal{T}_{b c}^{-1}\left[ \begin{matrix} \mathbf{R}_{w b}^{\top}\left(\mathbf{n}^{w}+\left[\mathbf{d}^{w}\right] \times \mathbf{p}_{wb}\right)\\ \mathbf{R}_{wb}^{\top}\mathbf{d}^w \end{matrix} \right]_{6 \times 1} \end{aligned} Lc=Tbc−1Twb−1Lw=Tbc−1[Rwb⊤(nw+[dw]×pwb)Rwb⊤dw]6×1
其中
T
b
c
=
[
R
b
c
[
p
b
c
]
×
R
b
c
0
R
b
c
]
T
b
c
−
1
=
[
R
b
c
⊤
−
R
b
c
⊤
[
p
b
c
]
×
0
R
b
c
⊤
]
\cal{T}_{bc} = \left[ \begin{array}{cc}{\mathbf{R}_{bc}} & {\left[\mathbf{p}_{bc }\right]_{\times} \mathbf{R}_{bc}} \\ {\mathbf{0}} & {\mathbf{R}_{bc}}\end{array}\right]\\ \cal{T}_{bc}^{-1} = \left[\begin{matrix} \bf{R}_{bc}^{\top} &- \bf{R}_{bc}^{\top} [p_{bc}]_{\times} \\0&\ \bf{R}_{bc}^{\top} \end{matrix}\right]
Tbc=[Rbc0[pbc]×RbcRbc]Tbc−1=[Rbc⊤0−Rbc⊤[pbc]× Rbc⊤]
−
[
a
]
×
b
=
[
b
]
×
a
-[a]_{\times}b=[b]_{\times}a
−[a]×b=[b]×a
线特征
L
\cal{L}
L 只优化状态变量中的位移和旋转,所以只需要对位移和旋转求导,其他都是零。下面我们来具体分析旋转和位移的求导。首先是线特征对旋转的求导:
∂ L c ∂ δ θ b b ′ = T b c − 1 [ ∂ ( I − [ δ θ b b ′ ] × ) R w b ⊤ ( n w + [ d w ] × p w b ) ∂ δ θ b b ′ ] ∂ ( I − [ δ θ b b ′ ] × ⊤ ) R w b ⊤ d w ∂ δ θ b b ′ ] = T b c − 1 [ [ R w b ⊤ ( n w + [ d w ] × p w b ) ] × ] [ R w b ⊤ d w ] × ] 6 × 3 \begin{align} \frac{\partial \mathcal{L}_{c}}{\partial \delta \theta_{b b^{\prime}}} &=\cal{T}_{bc}^{-1}\left[ \begin{array}{c}{\frac{\partial\left(\mathbf{I}-\left[\delta \boldsymbol{\theta}_{b b^{\prime}}\right]_\times\right) \mathbf{R}_{w b}^{\top}\left(\mathbf{n}^{w}+\left[\mathbf{d}^{w}\right]_\times \mathbf{p}_{w b}\right)}{\partial \delta \boldsymbol{\theta}_{b b^{\prime}}} ]} \\ {\frac{\partial\left(\mathbf{I}-\left[\delta \boldsymbol{\theta}_{b b^{\prime}}\right]_{\times}^{\top}\right) \mathbf{R}_{w b}^{\top} \mathbf{d}^{w}}{\partial \delta \boldsymbol{\theta}_{b b^{\prime}}}}\end{array}\right] \\ &=\mathcal{T}_{b c}^{-1} \left[ \begin{array}{c}{\left[\mathbf{R}_{w b}^{\top}\left(\mathbf{n}^{w}+\left[\mathbf{d}^{w}\right]_\times \mathbf{p}_{w b}\right)\right]_\times ]} \\ {\left[\mathbf{R}_{w b}^{\top} \mathbf{d}^{w}\right]_\times}\end{array}\right]_{6 \times 3} \end{align} ∂δθbb′∂Lc=Tbc−1 ∂δθbb′∂(I−[δθbb′]×)Rwb⊤(nw+[dw]×pwb)]∂δθbb′∂(I−[δθbb′]×⊤)Rwb⊤dw =Tbc−1[[Rwb⊤(nw+[dw]×pwb)]×][Rwb⊤dw]×]6×3
然后是线特征对位移的求导:
∂ L c ∂ δ p b b ′ = T b c − 1 [ ∂ R w b ⊤ ( n w + [ d w ] × ( p w b + δ p b b ′ ) ) ∂ δ p b b ′ ∂ R w b ⊤ d w ∂ δ p b b ′ ] = T b c − 1 [ R w b ⊤ [ d w ] × 0 ] 6 × 3 \begin{align} \frac{\partial\cal{L}_c}{\partial\delta \bf{p}_{bb^{\prime}}} &=\mathcal{T}_{b c}^{-1} \left[ \begin{array}{c}{\frac{\partial \mathbf{R}_{w b}^{\top}\left(\mathbf{n}^{w}+\left[\mathbf{d}^{w}\right]_{ \times}\left(\mathbf{p}_{w b}+\delta \mathbf{p}_{b b^{\prime}}\right)\right)}{\partial \delta \mathbf{p}_{b b^{\prime}}}} \\ {\frac{\partial \mathbf{R}_{w b}^{\top} \mathbf{d}^{w}}{\partial \delta \mathbf{p}_{b b^{\prime}}}}\end{array}\right] \\&=\mathcal{T}_{b c}^{-1} \left[ \begin{array}{c}{\mathbf{R}_{w b}^{\top}\left[\mathbf{d}^{w}\right]_{ \times}} \\ {0}\end{array}\right]_{6 \times 3} \end{align} ∂δpbb′∂Lc=Tbc−1 ∂δpbb′∂Rwb⊤(nw+[dw]×(pwb+δpbb′))∂δpbb′∂Rwb⊤dw =Tbc−1[Rwb⊤[dw]×0]6×3
第二部分中 ∂ L c i ∂ L w ∂ L w ∂ δ O \frac{\partial \mathcal{L}^{c_{i}}}{\partial \mathcal{L}^{w}} \frac{\partial \mathcal{L}^{w}}{\partial \delta \mathcal{O}} ∂Lw∂Lci∂δO∂Lw ,先解释第一个 ∂ L c i ∂ L w \frac{\partial \mathcal{L}^{c_{i}}}{\partial \mathcal{L}^{w}} ∂Lw∂Lci
L c = T w c − 1 L w \mathcal{L}^c = \mathcal{T}_{wc}^{-1}\mathcal{L}^w Lc=Twc−1Lw
所以 ∂ L c i ∂ L w = T w c − 1 \frac{\partial\cal{L}^{c_i}}{\partial\cal{L}^w} = \mathcal{T}_{wc}^{-1} ∂Lw∂Lci=Twc−1
然后后面的 ∂ L w ∂ δ O \frac{\partial \mathcal{L}^{w}}{\partial \delta \mathcal{O}} ∂δO∂Lw 有两种思路,先介绍第一种:
∂ L w ∂ δ O = [ ∂ L w ∂ ψ 1 ∂ L w ∂ ψ 2 ∂ L w ∂ ψ 3 ∂ L w ∂ ϕ ] ∂ L w ∂ ψ 1 = ∂ L w ∂ U ∂ U ∂ ψ 1 ∂ L w ∂ ϕ = ∂ L w ∂ w ∂ w ∂ ϕ 1 \frac{\partial \mathcal{L}^{w}}{\partial \delta \mathcal{O}} = \left[\begin{matrix} \frac{\partial\cal{L}^w}{\partial \psi_1} & \frac{\partial\cal{L}^w}{\partial \psi_2} & \frac{\partial\cal{L}^w}{\partial \psi_3} & \frac{\partial\cal{L}^w}{\partial \phi} \end{matrix} \right] \\ \frac{\partial \cal{L}^w}{\partial\psi_1} = \frac{\partial\cal{L}^w}{\partial \bf{U}}\frac{\partial \bf{U}}{\partial\psi_1} \\ \frac{\partial \cal{L}^w}{\partial\phi} = \frac{\partial\cal{L}^w}{\partial \bf{w}}\frac{\partial \bf{w}}{\partial\phi_1} ∂δO∂Lw=[∂ψ1∂Lw∂ψ2∂Lw∂ψ3∂Lw∂ϕ∂Lw]∂ψ1∂Lw=∂U∂Lw∂ψ1∂U∂ϕ∂Lw=∂w∂Lw∂ϕ1∂w
其中 L \cal{L} L 对 U \bf{U} U 和 w = [ w 1 , w 2 ] \mathbf{w}=[w_1,w_2] w=[w1,w2] 求导,因为 L w = [ w 1 u 1 ⊤ w 2 u 2 ⊤ ] ⊤ \mathcal{L}^w = \left[ \begin{matrix} w_1\bf{u}^{\top}_1&w_2\bf{u}^{\top}_2 \end{matrix}\right]^{\top} Lw=[w1u1⊤w2u2⊤]⊤ ,所以
∂ L ∂ U = [ ∂ L ∂ U 1 ∂ L ∂ U 2 ∂ L ∂ U 3 ] 6 × 9 = [ w 1 ( 3 × 3 ) 0 0 0 w 2 ( 3 × 3 ) 0 ] \begin{align} \frac{\partial\cal{L}}{\partial\bf{U}} &= \left[\begin{matrix} \frac{\partial\cal{L}}{\partial\bf{U}_1} & \frac{\partial\cal{L}}{\partial\bf{U}_2} & \frac{\partial\cal{L}}{\partial\bf{U}_3} \end{matrix}\right]_{6\times9} \\ &=\left[\begin{matrix} w_{1(3\times3)}&0&0\\0&w_{2(3\times3)}&0\end{matrix}\right] \end{align} ∂U∂L=[∂U1∂L∂U2∂L∂U3∂L]6×9=[w1(3×3)00w2(3×3)00]
∂ L ∂ w = [ ∂ L ∂ w 1 ∂ L ∂ w 2 ] 6 × 2 = [ u 1 0 0 u 2 ] \begin{align} \frac{\partial\cal{L}}{\partial\bf{w}} &= \left[\begin{matrix} \frac{\partial\cal{L}}{\partial w_1} & \frac{\partial\cal{L}}{\partial w_2} \end{matrix}\right]_{6\times2} \\ &=\left[\begin{matrix}\bf{u}_1&0 \\0&\bf{u}_2 \end{matrix}\right] \end{align} ∂w∂L=[∂w1∂L∂w2∂L]6×2=[u100u2]
然后是 U \bf{U} U 对 ψ \psi ψ 和 W \bf{W} W 对 ϕ \phi ϕ 的求导,
因为 U ′ ≈ U ( I + [ δ ψ ] × ) \begin{aligned} \mathbf{U}^{\prime} & \approx \mathbf{U}\left(\mathbf{I}+[\delta \psi]_{ \times}\right) \end{aligned} U′≈U(I+[δψ]×) ,所以
[ u 1 u 2 u 3 ] ′ = [ u 1 u 2 u 3 ] + [ u 1 u 2 u 3 ] × δ ψ [ u 1 u 2 u 3 ] ′ − [ u 1 u 2 u 3 ] δ ψ = [ u 1 u 2 u 3 ] × ∂ U ∂ ψ 1 = [ 0 u 3 − u 2 ] ∂ U ∂ ψ 2 = [ − u 3 0 u 1 ] ∂ U ∂ ψ 1 = [ u 2 − u 1 0 ] ∂ w ∂ ϕ = [ − w 2 w 1 ] \left[\begin{matrix} \bf{u}_1&\bf{u}_2 & \bf{u}_3 \end{matrix}\right]^{\prime} = \left[\begin{matrix} \bf{u}_1&\bf{u}_2 & \bf{u}_3 \end{matrix}\right] + \left[\begin{matrix} \bf{u}_1&\bf{u}_2 & \bf{u}_3 \end{matrix}\right]_{\times}\delta\psi \\\frac{ \left[\begin{matrix} \bf{u}_1&\bf{u}_2 & \bf{u}_3 \end{matrix}\right]^{\prime} - \left[\begin{matrix} \bf{u}_1&\bf{u}_2 & \bf{u}_3 \end{matrix}\right]}{\delta\psi} = \left[\begin{matrix} \bf{u}_1&\bf{u}_2 & \bf{u}_3 \end{matrix}\right]_{\times}\\ \frac{\partial\bf{U}}{\partial\psi_1} = \left[\begin{matrix} 0&\bf{u}_3 & -\bf{u}_2 \end{matrix}\right]\\ \frac{\partial\bf{U}}{\partial\psi_2} = \left[\begin{matrix} -\bf{u}_3&0 & \bf{u}_1 \end{matrix}\right]\\ \frac{\partial\bf{U}}{\partial\psi_1} = \left[\begin{matrix} \bf{u}_2 & -\bf{u}_1&0 \end{matrix}\right]\\ \frac{\partial\bf{w}}{\partial\phi} = \left[\begin{matrix} -w_2\\w_1 \end{matrix}\right] [u1u2u3]′=[u1u2u3]+[u1u2u3]×δψδψ[u1u2u3]′−[u1u2u3]=[u1u2u3]×∂ψ1∂U=[0u3−u2]∂ψ2∂U=[−u30u1]∂ψ1∂U=[u2−u10]∂ϕ∂w=[−w2w1]
所以,可得
∂ L w ∂ δ O = [ ∂ L w ∂ ψ 1 ∂ L w ∂ ψ 2 ∂ L w ∂ ψ 3 ∂ L w ∂ ϕ ] = [ ∂ L w ∂ U ∂ U ∂ ψ 1 ∂ L w ∂ U ∂ U ∂ ψ 2 ∂ L w ∂ U ∂ U ∂ ψ 3 ∂ L w ∂ w ∂ w ∂ ϕ ] = [ 0 − w 1 u 3 w 1 u 2 − w 2 u 1 w 2 u 3 0 − w 2 u 1 w 1 u 2 ] 6 × 4 \begin{align} \frac{\partial \mathcal{L}^{w}}{\partial \delta \mathcal{O}} &= \left[\begin{matrix} \frac{\partial\cal{L}^w}{\partial \psi_1} & \frac{\partial\cal{L}^w}{\partial \psi_2} & \frac{\partial\cal{L}^w}{\partial \psi_3} & \frac{\partial\cal{L}^w}{\partial \phi} \end{matrix} \right] \\ &= \left[\begin{matrix} \frac{\partial\cal{L}^w}{\partial\bf{U}}\frac{\partial\bf{U}}{\partial \psi_1} & \frac{\partial\cal{L}^w}{\partial\bf{U}}\frac{\partial\bf{U}}{\partial \psi_2} & \frac{\partial\cal{L}^w}{\partial\bf{U}}\frac{\partial\bf{U}}{\partial \psi_3} & \frac{\partial\cal{L}^w}{\partial \bf{w}}\frac{\partial \bf{w}}{\partial \phi} \end{matrix} \right] \\ &=\left[\begin{matrix}0&-w_1\bf{u}_3&w_1\bf{u}_2&-w_2\bf{u}_1\\w_2\bf{u}_3 &0&-w_2\bf{u}_1&w_1\bf{u}_2 \end{matrix} \right]_{6\times4} \end{align} ∂δO∂Lw=[∂ψ1∂Lw∂ψ2∂Lw∂ψ3∂Lw∂ϕ∂Lw]=[∂U∂Lw∂ψ1∂U∂U∂Lw∂ψ2∂U∂U∂Lw∂ψ3∂U∂w∂Lw∂ϕ∂w]=[0w2u3−w1u30w1u2−w2u1−w2u1w1u2]6×4
4.3.误差雅可比求导简洁版(不含imu坐标系转换)
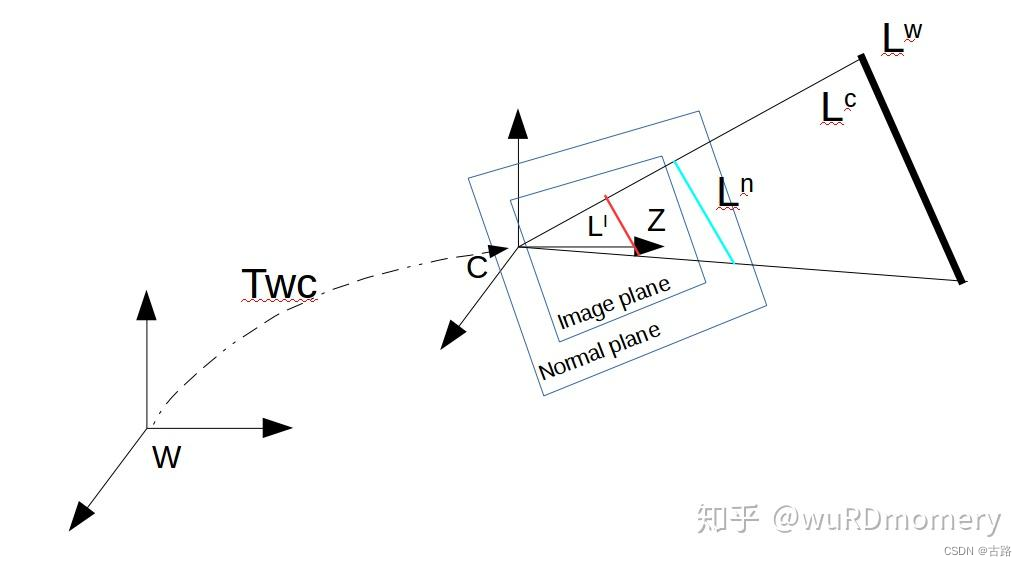
- L W L^W LW表示在世界坐标系的表示, L C L^{C} LC表示在相机坐标系下的表示;
- L n L^n Ln表示归一化平面上的线, L I L^I LI表示在图像坐标系下的线;
图中的 I L I_L IL表示直线 L \mathcal{L} L在图像平面的投影,所以定义误差项为(就是简单的两个点到直线的距离):
r L = [ r 1 r 2 ] = [ c T I l 1 2 + l 2 2 d T I l 1 2 + l 2 2 ] (15) \mathbf{r_L}=\begin{bmatrix}\mathbf{r_1} \\ \mathbf{r_2} \end{bmatrix} = \begin{bmatrix}\frac{c^TI}{\sqrt{l_1^2+l_2^2}} \\ \frac{d^TI}{\sqrt{l_1^2+l_2^2}} \end{bmatrix} \tag{15} rL=[r1r2]= l12+l22cTIl12+l22dTI (15)
求解Jacobian
跟对3D点的优化问题一样,就是从误差不停的递推到位姿以及直线表示上,用到最最最基本的求导的链式法则:
通用的公式如下:
∂ r L ∂ X = ∂ r L ∂ L I ∂ L I ∂ L n ∂ L n ∂ L c { ∂ L c ∂ θ X= θ ∂ L c ∂ t X=t ∂ L c ∂ L w ∂ L w ∂ ( θ , ϕ ) X= L w (16) \frac{\partial \mathbf{r_L}}{\partial X}= \frac{\partial \mathbf{r_L}}{\partial L^{I}} \frac{\partial L^{I}}{\partial L^{n}} \frac{\partial L^{n}}{\partial L^{c}} \begin{aligned} \begin{cases} \frac{\partial L^{c}}{\partial \theta} &\text{ X=}\theta \\ \frac{\partial L^{c}}{\partial t} &\text{ X=t} \\ \frac{\partial L^{c}}{\partial L^{w}}\frac{\partial L^{w}}{\partial{(\theta,\phi)}} &\text{ X=}L^{w} \end{cases} \end{aligned}\tag{16} ∂X∂rL=∂LI∂rL∂Ln∂LI∂Lc∂Ln⎩ ⎨ ⎧∂θ∂Lc∂t∂Lc∂Lw∂Lc∂(θ,ϕ)∂Lw X=θ X=t X=Lw(16)
先对前面最通用的部分进行求解:
第一部分:
∂ r L ∂ L I = [ ∂ r 1 ∂ l 1 ∂ r 1 ∂ l 2 ∂ r 1 ∂ l 3 ∂ r 2 ∂ l 1 ∂ r 2 ∂ l 2 ∂ r 2 ∂ l 3 ] = [ − l 1 c T L I ( l 1 2 + l 2 2 ) 3 2 + u c ( l 1 2 + l 2 2 ) 1 2 − l 2 c T L I ( l 1 2 + l 2 2 ) 3 2 + v c ( l 1 2 + l 2 2 ) 1 2 1 ( l 1 2 + l 2 2 ) 1 2 − l 1 d T L I ( l 1 2 + l 2 2 ) 3 2 + u d ( l 1 2 + l 2 2 ) 1 2 − l 2 d T L I ( l 1 2 + l 2 2 ) 3 2 + v d ( l 1 2 + l 2 2 ) 1 2 1 ( l 1 2 + l 2 2 ) 1 2 ] 2 × 3 (17) \begin{aligned} \frac{\partial \mathbf{r_L}}{\partial L^{I}} &= \begin{bmatrix}\frac{\partial{\mathbf{r1}}}{\partial{l_1}} & \frac{\partial{\mathbf{r1}}}{\partial{l_2}} & \frac{\partial{\mathbf{r1}}}{\partial{l_3}} \\ \frac{\partial{\mathbf{r2}}}{\partial{l_1}} & \frac{\partial{\mathbf{r2}}}{\partial{l_2}} & \frac{\partial{\mathbf{r2}}}{\partial{l_3}}\end{bmatrix} \\ &=\begin{bmatrix}\frac{-l_1 c^TL^{I}}{(l_1^2+l_2^2)^{\frac{3}{2}}}+\frac{u_c}{(l_1^2+l_2^2)^{\frac{1}{2}}} & \frac{-l_2 c^TL^{I}}{(l_1^2+l_2^2)^{\frac{3}{2}}}+\frac{v_c}{(l_1^2+l_2^2)^{\frac{1}{2}}} & \frac{1}{(l_1^2+l_2^2)^{\frac{1}{2}}} \\ \frac{-l_1 d^TL^{I}}{(l_1^2+l_2^2)^{\frac{3}{2}}}+\frac{u_d}{(l_1^2+l_2^2)^{\frac{1}{2}}} & \frac{-l_2 d^TL^{I}}{(l_1^2+l_2^2)^{\frac{3}{2}}}+\frac{v_d}{(l_1^2+l_2^2)^{\frac{1}{2}}} & \frac{1}{(l_1^2+l_2^2)^{\frac{1}{2}}} \end{bmatrix}_{2\times 3} \end{aligned} \tag{17} ∂LI∂rL=[∂l1∂r1∂l1∂r2∂l2∂r1∂l2∂r2∂l3∂r1∂l3∂r2]= (l12+l22)23−l1cTLI+(l12+l22)21uc(l12+l22)23−l1dTLI+(l12+l22)21ud(l12+l22)23−l2cTLI+(l12+l22)21vc(l12+l22)23−l2dTLI+(l12+l22)21vd(l12+l22)211(l12+l22)211 2×3(17)
其中:
l
1
,
l
2
,
l
3
l1, l2, l3
l1,l2,l3表示图像坐标系下直线的三个参数;
u
c
,
v
c
u_c, v_c
uc,vc表示点
c
c
c的
x
y
xy
xy坐标值,
u
d
,
v
d
u_d, v_d
ud,vd同理;
第二部分:
根据公式(13)可知:
∂ L I ∂ L n = K 3 × 3 (18) \frac{\partial L^{I}}{\partial L^{n}}=\mathcal{K}_{3\times3} \tag{18} ∂Ln∂LI=K3×3(18)
第三部分:
由公式(6)和(13)可知,直线的Plucker表示在归一化平面上只用了其中的法向量部分,因此若有 L c = [ n c , d c ] T \mathcal{L{^c}}=\left[\mathbf{n{^c}}, \mathbf{d{^c}}\right]^T Lc=[nc,dc]T,那么 L n = n c \mathcal{L{^n}}=\mathbf{n{^c}} Ln=nc,所以求导有:
∂ L n ∂ L c = [ I 3 × 3 0 3 × 3 ] 3 × 6 (19) \frac{\partial L^{n}}{\partial L^{c}}=\begin{bmatrix}\mathbf{I}_{3\times3} & 0_{3\times3}\end{bmatrix}_{3\times6} \tag{19} ∂Lc∂Ln=[I3×303×3]3×6(19)
第四部分就分这几种情况进行讨论:
对于位姿的姿态部分
根据公式(7)有:
∂ L c ∂ θ = [ ∂ n c ∂ θ ∂ d c ∂ θ ] = [ ∂ ( R w c T ( n w + [ t w c ] × b w ) ) ∂ θ ∂ R w c T b w ∂ θ ] = [ [ R w c T ( n w + [ t w c ] × b w ) ] × [ R w c T b w ] × ] 6 × 3 (20) \frac{\partial L{^c}}{\partial \theta} = \begin{bmatrix}\frac{\partial n_c}{\partial \theta} \\ \frac{\partial d_c}{\partial \theta}\end{bmatrix} = \begin{bmatrix}\frac{\partial{(R_{wc}^T(n_w+[t_{wc}]_{\times}b_w))}}{\partial \theta} \\ \frac{\partial{R_{wc}^Tb_w}}{\partial \theta}\end{bmatrix}=\begin{bmatrix} [R_{wc}^T(n_w+[t_{wc}]_{\times}b_w)]_{\times} \\ [R_{wc}^Tb_w]_{\times} \end{bmatrix}_{6\times3} \tag{20} ∂θ∂Lc=[∂θ∂nc∂θ∂dc]=[∂θ∂(RwcT(nw+[twc]×bw))∂θ∂RwcTbw]=[[RwcT(nw+[twc]×bw)]×[RwcTbw]×]6×3(20)
上述的推导使用了李群的右扰动模型,即 ( R w c E x p ( θ ) ) T = E x p ( − θ ) R w c T (R_{wc}Exp(\theta))^T=Exp(-\theta)R_{wc}^T (RwcExp(θ))T=Exp(−θ)RwcT。
对于位姿的位移部分
同样根据公式(7)有:
∂ L c ∂ t = [ ∂ n c ∂ t ∂ d c ∂ t ] = [ ∂ ( R w c T ( n w + [ t w c ] × b w ) ) ∂ t ∂ R w c T b w ∂ t ] = [ − R w c T [ b w ] × 0 ] 6 × 3 (21) \frac{\partial L{^c}}{\partial t} = \begin{bmatrix}\frac{\partial n_c}{\partial t} \\ \frac{\partial d_c}{\partial t}\end{bmatrix} = \begin{bmatrix}\frac{\partial{(R_{wc}^T(n_w+[t_{wc}]_{\times}b_w))}}{\partial t} \\ \frac{\partial{R_{wc}^Tb_w}}{\partial t}\end{bmatrix}=\begin{bmatrix} -R_{wc}^T[b_{w}]_{\times} \\ \mathbf{0} \end{bmatrix}_{6\times3} \tag{21} ∂t∂Lc=[∂t∂nc∂t∂dc]=[∂t∂(RwcT(nw+[twc]×bw))∂t∂RwcTbw]=[−RwcT[bw]×0]6×3(21)
对于世界坐标系下直线表示部分
这部分按照公式(16)的步骤,依旧分两个部分:
∂
L
c
∂
L
w
\frac{\partial L^{c}}{\partial L^{w}}
∂Lw∂Lc部分:
∂
L
c
∂
L
w
=
[
∂
n
C
∂
n
W
∂
n
C
∂
b
W
∂
d
C
∂
n
W
∂
d
C
∂
b
W
]
=
[
R
w
c
T
R
w
c
T
[
t
w
c
]
×
0
R
w
c
T
]
(22)
\frac{\partial L^{c}}{\partial L^{w}}= \begin{bmatrix} \frac{\partial{\mathbf{n^C}}}{\partial{\mathbf{n^W}}} & \frac{\partial{\mathbf{n^C}}}{\partial{\mathbf{b^W}}} \\ \frac{\partial{\mathbf{d^C}}}{\partial{\mathbf{n^W}}} & \frac{\partial{\mathbf{d^C}}}{\partial{\mathbf{b^W}}} \end{bmatrix} = \left[\begin{array}{cc} \mathrm{R}_{wc}^{T} & {\mathrm{R}_{wc}^{T}\left[\mathbf{t}_{wc}\right]_{\times} } \\ \mathbf{0} & \mathrm{R}_{wc}^T \end{array}\right] \tag{22}
∂Lw∂Lc=[∂nW∂nC∂nW∂dC∂bW∂nC∂bW∂dC]=[RwcT0RwcT[twc]×RwcT](22)
∂
L
w
∂
(
θ
,
ϕ
)
\frac{\partial L^{w}}{\partial(\theta, \phi)}
∂(θ,ϕ)∂Lw部分,这部分其实还可以继续分,如下:
∂
L
w
∂
(
θ
,
ϕ
)
=
[
∂
L
w
∂
θ
,
∂
L
w
∂
ϕ
]
=
[
∂
L
w
∂
U
∂
U
∂
θ
,
∂
L
w
∂
W
∂
W
∂
ϕ
]
\frac{\partial L^{w}}{\partial(\theta, \phi)}= \left[\frac{\partial L^{w}}{\partial \theta}, \frac{\partial L^{w}}{\partial \phi}\right]= \left[\frac{\partial L^{w}}{\partial{U}}\frac{\partial{U}}{\partial \theta}, \frac{\partial L^{w}}{\partial{W}}\frac{\partial{W}}{\partial \phi}\right]
∂(θ,ϕ)∂Lw=[∂θ∂Lw,∂ϕ∂Lw]=[∂U∂Lw∂θ∂U,∂W∂Lw∂ϕ∂W]第一部分
∂
L
w
∂
U
∂
U
∂
θ
=
∂
[
w
1
u
1
w
2
u
2
]
∂
[
u
1
,
u
2
,
u
3
]
∂
[
u
1
,
u
2
,
u
3
]
∂
θ
=
[
w
1
0
0
0
w
2
0
]
6
×
9
[
0
−
u
3
u
2
u
3
0
−
u
1
−
u
2
u
1
0
]
9
×
3
=
[
0
−
w
1
u
3
w
1
u
2
−
w
2
u
3
0
−
w
2
u
1
]
6
×
3
(23)
\begin{aligned} \frac{\partial L^{w}}{\partial{U}}\frac{\partial{U}}{\partial \theta}&=\frac{\partial{\begin{bmatrix} w1\mathbf{u_1} \\ w2\mathbf{u_2} \end{bmatrix}}}{\partial{[\mathbf{u_1},\mathbf{u_2}, \mathbf{u_3}]}}\frac{\partial{[\mathbf{u_1},\mathbf{u_2}, \mathbf{u_3}]}}{\partial{\theta}} \\ &=\begin{bmatrix}w1 & 0 & 0 \\ 0 & w2 & 0 \end{bmatrix}_{6\times9} \begin{bmatrix}0 & -\mathbf{u3} & \mathbf{u2} \\ \mathbf{u3} & 0 & -\mathbf{u1} \\ -\mathbf{u2} & \mathbf{u1} & 0 \end{bmatrix}_{9\times3} \\ &= \begin{bmatrix} 0 & -w1\mathbf{u3} & w1\mathbf{u2} \\ -w2\mathbf{u3} & 0 & -w2\mathbf{u1} \end{bmatrix}_{6\times3} \end{aligned} \tag{23}
∂U∂Lw∂θ∂U=∂[u1,u2,u3]∂[w1u1w2u2]∂θ∂[u1,u2,u3]=[w100w200]6×9
0u3−u2−u30u1u2−u10
9×3=[0−w2u3−w1u30w1u2−w2u1]6×3(23) 第二部分
∂
L
w
∂
W
∂
W
∂
ϕ
=
∂
[
w
1
u
1
w
2
u
2
]
∂
[
w
1
,
w
2
]
T
∂
[
w
1
,
w
2
]
T
∂
ϕ
=
[
u
1
0
0
u
2
]
6
×
2
[
−
w
2
w
1
]
2
×
1
=
[
−
w
2
u
1
w
1
u
2
]
6
×
1
(24)
\begin{aligned} \frac{\partial L^{w}}{\partial{W}}\frac{\partial{W}}{\partial \phi}&=\frac{\partial{\begin{bmatrix} w1\mathbf{u_1} \\ w2\mathbf{u_2} \end{bmatrix}}}{\partial{[w1, w2]^T}}\frac{\partial{[w1, w2]^T}}{\partial{\phi}} \\ &=\begin{bmatrix}\mathbf{u1} & 0 \\ 0 & \mathbf{u2} \end{bmatrix}_{6\times2} \begin{bmatrix} -w2 \\ w1 \end{bmatrix}_{2\times1} \\ &= \begin{bmatrix} -w2\mathbf{u1} \\ w1\mathbf{u2}\end{bmatrix}_{6\times1} \end{aligned} \tag{24}
∂W∂Lw∂ϕ∂W=∂[w1,w2]T∂[w1u1w2u2]∂ϕ∂[w1,w2]T=[u100u2]6×2[−w2w1]2×1=[−w2u1w1u2]6×1(24) 其中
w
1
=
c
o
s
(
ϕ
)
,
w
2
=
s
i
n
(
ϕ
)
w1=cos(\phi), w2=sin(\phi)
w1=cos(ϕ),w2=sin(ϕ)。
两个部分合起来为:
∂
L
w
∂
(
θ
,
ϕ
)
=
[
R
w
c
T
R
w
c
T
[
t
w
c
]
×
0
R
w
c
T
]
6
×
6
[
0
−
w
1
u
3
w
1
u
2
−
w
2
u
1
−
w
2
u
3
0
−
w
2
u
1
w
1
u
2
]
6
×
4
(25)
\frac{\partial L^{w}}{\partial(\theta, \phi)}= \left[\begin{array}{cc} \mathrm{R}_{wc}^{T} & {\mathrm{R}_{wc}^{T}\left[\mathbf{t}_{wc}\right]_{\times} } \\ \mathbf{0} & \mathrm{R}_{wc}^T \end{array}\right]_{6\times6} \begin{bmatrix} 0 & -w1\mathbf{u3} & w1\mathbf{u2} & -w2\mathbf{u1} \\ -w2\mathbf{u3} & 0 & -w2\mathbf{u1} & w1\mathbf{u2} \end{bmatrix}_{6\times4} \tag{25}
∂(θ,ϕ)∂Lw=[RwcT0RwcT[twc]×RwcT]6×6[0−w2u3−w1u30w1u2−w2u1−w2u1w1u2]6×4(25)
最后就是上述推导过程中确实有很多地方向量的notation没有统一,可能有些比较容易混淆,这里确实是因为各个论文的表示不太一样,导致写公式的时候不太一样,自己又偷了个懒,不过该注释的地方都进行了注释。目前比较流行的表示应该是 L = [ n , d ] T L=[\mathbf{n}, \mathbf{d}]^T L=[n,d]T 或者 L = [ n , v ] T L=[\mathbf{n}, \mathbf{v}]^T L=[n,v]T ,其中 n \mathbf{n} n 表示法向量, d \mathbf{d} d 或者 v \mathbf{v} v 表示方向向量。
4.4.相关代码
优化入口,ceres,主要实现在这里。
- todo:导数填充