论文:TPAMI 2023 图神经网络的强化因果解释器
论文代码地址:代码
目录
Abstract
Introduction
PRELIMINARIES
Causal Attribution of a Holistic Subgraph
individual causal effect (ICE)
*Causal Screening of an Edge Sequence
Reinforced Causal Explainer (RC-Explainer)
Policy Network
Policy Gradient Training
Discussion
EXPERIMENTS
Evaluation Metrics
Evaluation of Explanations
Abstract
Motivation:解释图神经网络(GNNs)的预测结果来理解模型决策背后的原因。现有Feature attribution忽略了边之间的依赖关系,尤其是协同效应。
Method:引入Reinforced Causal Explainer(RC-Explainer)实现因果筛选策略, 策略网络学习边序列生成策略(每个边缘被选中的概率),在每step选择一个潜在边缘作为action,获得由每个边的组合和子图的因果属性组成的reward,可突出解释边的依赖性、边的联盟的影响。
用策略梯度来优化策略网络,并通过对GNN全局理解,RC-Explainer能为每个图实例提供模型级解释,并泛化到未见过的图。
Conclusion:在解释三个图分类数据集上不同的GNN时,RC-Explainer在predictive accuracy、contrastivity等两个定量指标上实现了与最先进方法相当或更好的性能,并通过了合理性检查(sanity checks)和视觉检查(visual inspections)。
一、Introduction
PRELIMINARIES
相关代码实现:Mutag_gnn.py
节点表示:
#获取节点表示
def get_node_reps(self, x, edge_index, edge_attr, batch):
node_x = self.node_emb(x)#节点嵌入层
edge_attr = self.edge_emb(edge_attr)#边嵌入层
# 对于每个 GINConv 单元
for conv, batch_norm, ReLU in \
zip(self.convs, self.batch_norms, self.relus):
node_x = conv(node_x, edge_index, edge_attr) #节点表示传递给GINConv层进行信息聚合
node_x = ReLU(batch_norm(node_x))#标准化,激活函数
return node_x
最终用于预测的表示:
def get_graph_rep(self, x, edge_index, edge_attr, batch):
node_x = self.get_node_reps(x, edge_index, edge_attr, batch)
graph_x = global_mean_pool(node_x, batch)
return graph_x
def get_pred(self, graph_x):
pred = self.relu(self.lin1(graph_x))#线性层,relu处理图表示
pred = self.lin2(pred)#预测
self.readout = self.softmax(pred)
return pred
Causal Attribution of a Holistic Subgraph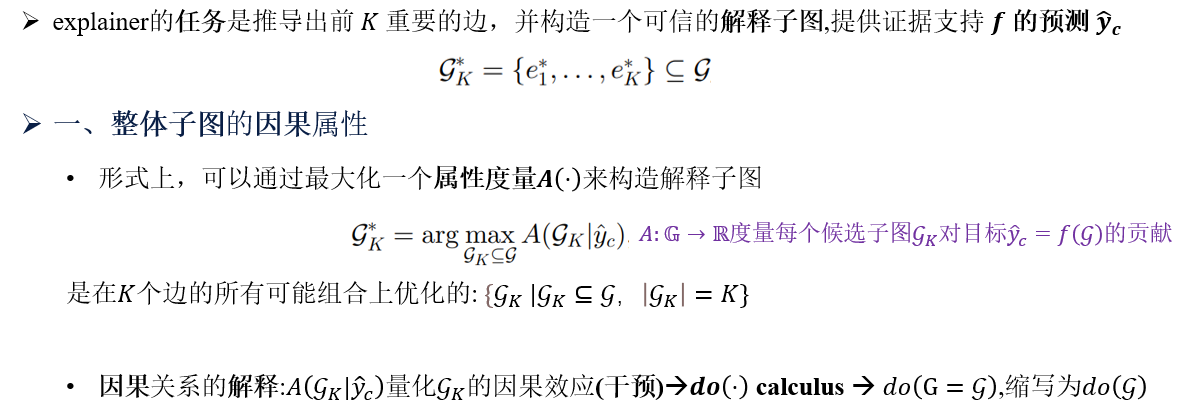
individual causal effect (ICE)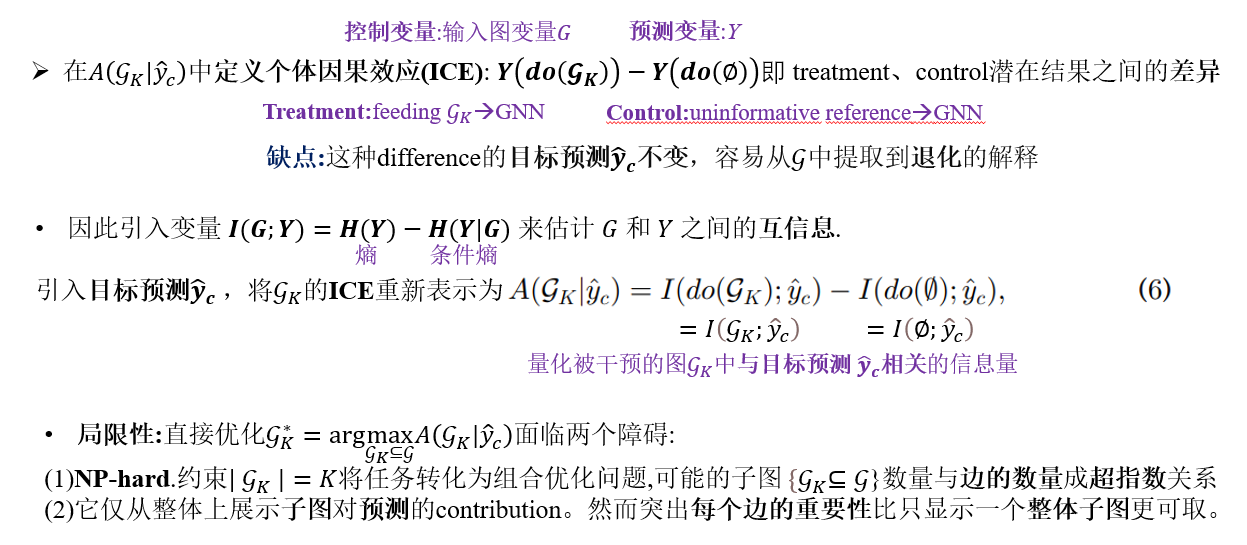
论文代码中对于互信息的实现,在reward的计算中
def get_reward(full_subgraph_pred, new_subgraph_pred, target_y, pre_reward, mode='mutual_info'):
if mode in ['mutual_info']:
#计算互信息,衡量完整子图预测值和新子图预测值之间的相似度
# full_subgraph_pred:[batch_size, num_classes] reward:[batch_size]
reward = torch.sum(full_subgraph_pred * torch.log(new_subgraph_pred + EPS), dim=1)
#对每个样本,新子图预测的最大类别与目标类别相同+1;否则-1
reward += 2 * (target_y == new_subgraph_pred.argmax(dim=1)).float() - 1.
# print('reward2',reward)
elif mode in ['binary']:
# 新子图预测的最大类别与目标类别相同,奖励+1;否则-1
reward = (target_y == new_subgraph_pred.argmax(dim=1)).float()
reward = 2. * reward - 1.
elif mode in ['cross_entropy']:
# 交叉熵作为奖励,衡量完整子图预测值与目标类别之间的差异
reward = torch.log(new_subgraph_pred + EPS)[:, target_y]
# reward += pre_reward
reward += 0.97 * pre_reward
return reward
*Causal Screening of an Edge Sequence
Reinforced Causal Explainer (RC-Explainer)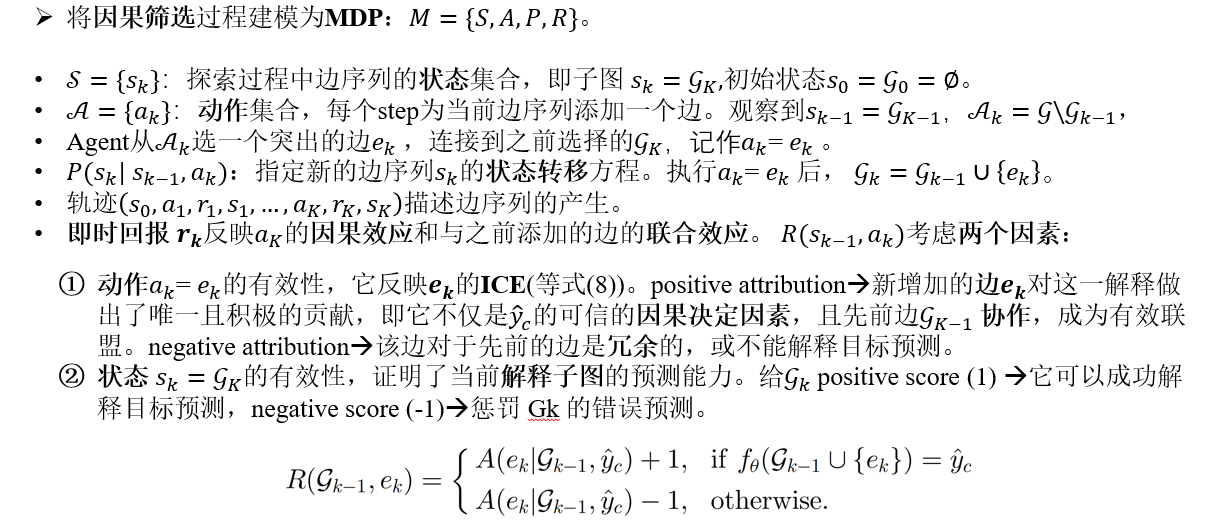
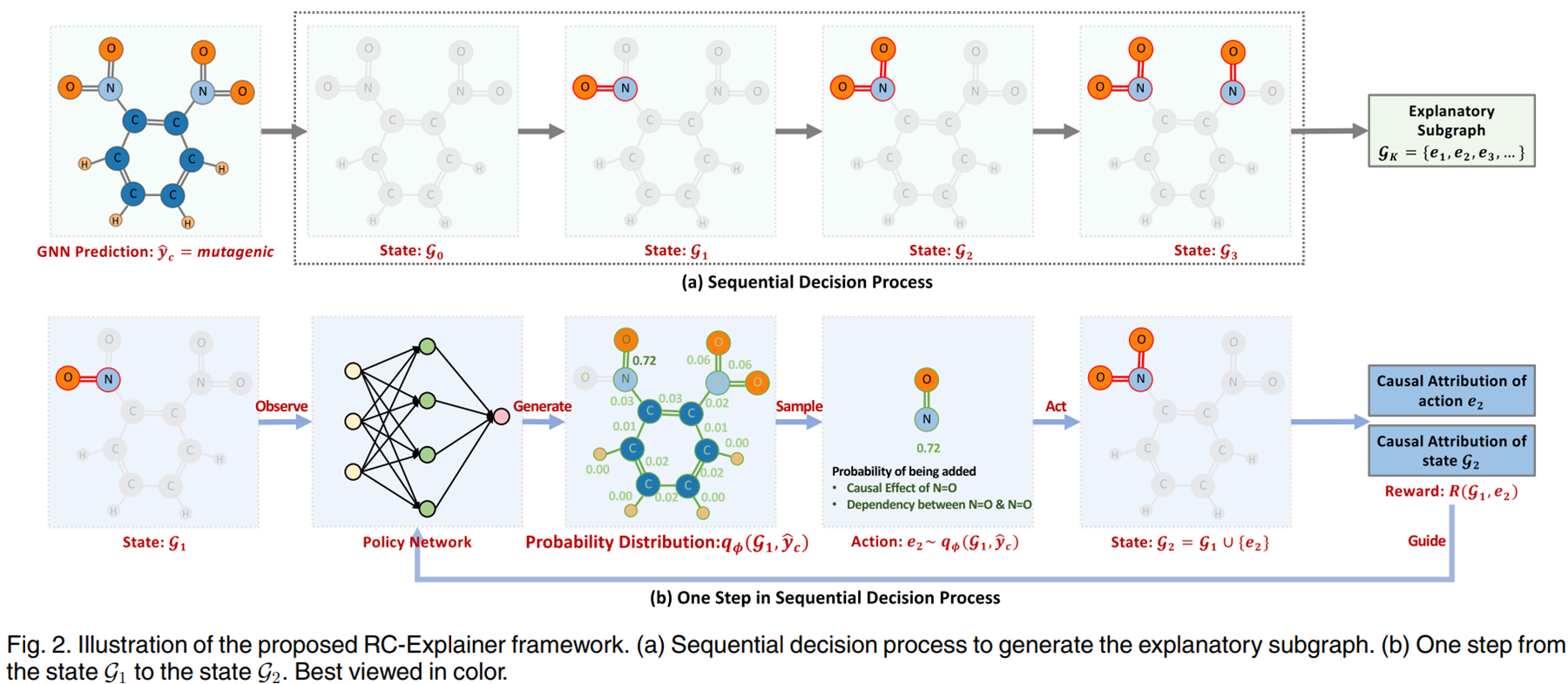
主要流程框架:train_test_pool_batch3.py
def test_policy_all_with_gnd(rc_explainer, model, test_loader, topN=None):
rc_explainer.eval()
model.eval()
topK_ratio_list = [0.1, 0.2, 0.3, 0.4, 0.5, 0.6, 0.7, 0.8, 0.9, 1.0]
acc_count_list = np.zeros(len(topK_ratio_list))
precision_topN_count = 0.
recall_topN_count = 0.
with torch.no_grad():
for graph in iter(test_loader):
graph = graph.to(device)
max_budget = graph.num_edges#最大预算
state = torch.zeros(max_budget, dtype=torch.bool)#当前状态
# 根据 top K 比率列表计算出需要检查准确率的预算列表
check_budget_list = [max(int(_topK * max_budget), 1) for _topK in topK_ratio_list]
valid_budget = max(int(0.9 * max_budget), 1)#有效预算
for budget in range(valid_budget):#每一个预算
available_actions = state[~state].clone()#可用的动作
# 获取下一步的动作
_, _, make_action_id, _ = rc_explainer(graph=graph, state=state, train_flag=False)
# 将推断的动作应用到可用动作列表中
available_actions[make_action_id] = True
state[~state] = available_actions.clone()#更新当前状态
# 如果当前预算需要检查准确率
if (budget + 1) in check_budget_list:
check_idx = check_budget_list.index(budget + 1)#查找当前预算在 check_budget_list 中的索引
subgraph = relabel_graph(graph, state)
# 用模型对子图进行预测
subgraph_pred = model(subgraph.x, subgraph.edge_index, subgraph.edge_attr, subgraph.batch)
# 计算准确率并累加到对应的位置
acc_count_list[check_idx] += sum(graph.y == subgraph_pred.argmax(dim=1))
print('graph.ground_truth_mask[0]',graph.ground_truth_mask[0])
# 指定了 topN & 当前预算=topN-1
if topN is not None and budget == topN - 1:
print('graph.ground_truth_mask[0]',graph.ground_truth_mask[0])
# 累加前N个动作的精度
precision_topN_count += torch.sum(state*graph.ground_truth_mask[0])/topN
recall_topN_count += torch.sum(state*graph.ground_truth_mask[0])/sum(graph.ground_truth_mask[0])
acc_count_list[-1] = len(test_loader)
acc_count_list = np.array(acc_count_list)/len(test_loader)
precision_topN_count = precision_topN_count / len(test_loader)
recall_topN_count = recall_topN_count / len(test_loader)
if topN is not None:
print('\nACC-AUC: %.4f, Precision@5: %.4f, Recall@5: %.4f' %
(acc_count_list.mean(), precision_topN_count, recall_topN_count))
else:
print('\nACC-AUC: %.4f' % acc_count_list.mean())
print(acc_count_list)
return acc_count_list.mean(), acc_count_list, precision_topN_count, recall_topN_count
其中这四步的实现: rc_explainer_pool.py
class RC_Explainer_Batch_star(RC_Explainer_Batch):
def __init__(self, _model, _num_labels, _hidden_size, _use_edge_attr=False):
super(RC_Explainer_Batch_star, self).__init__(_model, _num_labels, _hidden_size, _use_edge_attr=False)
# 单层MLP
def build_edge_action_prob_generator(self):
edge_action_prob_generator = nn.ModuleList()
for i in range(self.num_labels):
i_explainer = Sequential(
Linear(self.hidden_size * (2 + self.use_edge_attr), self.hidden_size * 2),
ELU(),
Linear(self.hidden_size * 2, self.hidden_size),
ELU(),
Linear(self.hidden_size, 1)
).to(device)
edge_action_prob_generator.append(i_explainer)
return edge_action_prob_generator
def forward(self, graph, state, train_flag=False):
#整个图表示 graph_rep-->torch.Size([64, 32])
graph_rep = self.model.get_graph_rep(graph.x, graph.edge_index, graph.edge_attr, graph.batch)
#若不存在已使用的边,创建全0子图表示
if len(torch.where(state==True)[0]) == 0:
subgraph_rep = torch.zeros(graph_rep.size()).to(device)
else:
subgraph = relabel_graph(graph, state)#根据状态重新标记图
subgraph_rep = self.model.get_graph_rep(subgraph.x, subgraph.edge_index, subgraph.edge_attr, subgraph.batch)
# 可用边索引、属性
ava_edge_index = graph.edge_index.T[~state].T #torch.Size([2, 3666])
ava_edge_attr = graph.edge_attr[~state]#torch.Size([3362, 3])
#未使用边对应的节点表示->torch.Size([2153, 32])
ava_node_reps = self.model.get_node_reps(graph.x, ava_edge_index, ava_edge_attr, graph.batch)
# 学习每个候选动作表示
if self.use_edge_attr:#使用边属性信息,将未使用边嵌入可用边表示
ava_edge_reps = self.model.edge_emb(ava_edge_attr)
ava_action_reps = torch.cat([ava_node_reps[ava_edge_index[0]],
ava_node_reps[ava_edge_index[1]],
ava_edge_reps], dim=1).to(device)
else:
ava_action_reps = torch.cat([ava_node_reps[ava_edge_index[0]],
ava_node_reps[ava_edge_index[1]]], dim=1).to(device)#torch.Size([3824, 64])
#边动作表示生成器
ava_action_reps = self.edge_action_rep_generator(ava_action_reps)#torch.Size([3760, 32])
#未使用边所属图
ava_action_batch = graph.batch[ava_edge_index[0]]#[ 0, 0, 0, ..., 63, 63, 63] torch.Size([4016])
#图标签
ava_y_batch = graph.y[ava_action_batch]#[0, 0, 0, ..., 1, 1, 1] torch.Size([3794])
# get the unique elements in batch, in cases where some batches are out of actions.
unique_batch, ava_action_batch = torch.unique(ava_action_batch, return_inverse=True)#[64],[3760]
#选择一个动作,预测未使用的边的动作概率
ava_action_probs = self.predict_star(graph_rep, subgraph_rep, ava_action_reps, ava_y_batch, ava_action_batch)
# print(ava_action_probs,ava_action_probs.size())
# assert len(ava_action_probs) == sum(~state)
#每个图中最大概率及动作
added_action_probs, added_actions = scatter_max(ava_action_probs, ava_action_batch)
if train_flag:#训练
rand_action_probs = torch.rand(ava_action_probs.size()).to(device)# 生成一个与未使用的边的动作概率相同大小的随机概率张量
#每个图中最大的随机概率动作
_, rand_actions = scatter_max(rand_action_probs, ava_action_batch)
return ava_action_probs, ava_action_probs[rand_actions], rand_actions, unique_batch
return ava_action_probs, added_action_probs, added_actions, unique_batch
def predict_star(self, graph_rep, subgraph_rep, ava_action_reps, target_y, ava_action_batch):
action_graph_reps = graph_rep - subgraph_rep#可用图表示
action_graph_reps = action_graph_reps[ava_action_batch]#索引可用图表示
#未使用边动作表示拼接动作图表示->完整的动作表示
action_graph_reps = torch.cat([ava_action_reps, action_graph_reps], dim=1)
action_probs = []
for i_explainer in self.edge_action_prob_generator:#对于每个标签的动作解释器
i_action_probs = i_explainer(action_graph_reps)#当前标签的动作解释器预测动作概率
action_probs.append(i_action_probs)
action_probs = torch.cat(action_probs, dim=1)#每个标签的动作概率连接,每一列->一个标签的动作概率
#从预测的动作概率中索引标签对应的概率
action_probs = action_probs.gather(1, target_y.view(-1,1))
action_probs = action_probs.reshape(-1)#一维
# action_probs = softmax(action_probs, ava_action_batch)
# action_probs = F.sigmoid(action_probs)
return action_probs
Policy Network
论文相关代码实现:rc_explainer_pool.py RC_Explainer_Batch_star()
ava_node_reps = self.model.get_node_reps(graph.x, ava_edge_index, ava_edge_attr, graph.batch)
# 学习每个候选动作表示
if self.use_edge_attr:#使用边属性信息,将未使用边嵌入可用边表示
ava_edge_reps = self.model.edge_emb(ava_edge_attr)
ava_action_reps = torch.cat([ava_node_reps[ava_edge_index[0]],
ava_node_reps[ava_edge_index[1]],
ava_edge_reps], dim=1).to(device)
else:
ava_action_reps = torch.cat([ava_node_reps[ava_edge_index[0]],
ava_node_reps[ava_edge_index[1]]], dim=1).to(device)#torch.Size([3824, 64])
#边动作表示生成器
ava_action_reps = self.edge_action_rep_generator(ava_action_reps)#torch.Size([3760, 32])
论文相关代码实现:rc_explainer_pool.py
def predict_star(self, graph_rep, subgraph_rep, ava_action_reps, target_y, ava_action_batch):
action_graph_reps = graph_rep - subgraph_rep#可用图表示
action_graph_reps = action_graph_reps[ava_action_batch]#索引可用图表示
#未使用边动作表示拼接动作图表示->完整的动作表示
action_graph_reps = torch.cat([ava_action_reps, action_graph_reps], dim=1)
action_probs = []
for i_explainer in self.edge_action_prob_generator:#对于每个标签的动作解释器
i_action_probs = i_explainer(action_graph_reps)#当前标签的动作解释器预测动作概率
action_probs.append(i_action_probs)
action_probs = torch.cat(action_probs, dim=1)#每个标签的动作概率连接,每一列->一个标签的动作概率
#从预测的动作概率中索引标签对应的概率
action_probs = action_probs.gather(1, target_y.view(-1,1))
action_probs = action_probs.reshape(-1)#一维
# action_probs = softmax(action_probs, ava_action_batch)
# action_probs = F.sigmoid(action_probs)
return action_probs
Policy Gradient Training
论文相关代码实现:train_test_pool_batch3.py train_policy()
# 批次损失(RL REINFORCE策略梯度)
batch_loss += torch.mean(- torch.log(beam_action_probs_list + EPS) * beam_reward_list)
Discussion
EXPERIMENTS
Evaluation Metrics
论文相关代码实现:一、ACC train_test_pool_batch3.py test_policy_all_with_gnd()
# 计算准确率并累加到对应的位置
acc_count_list[check_idx] += sum(graph.y == subgraph_pred.argmax(dim=1))