1 从明暗恢复形状
从明暗恢复形状(Shape from Shading,SfS)是指从图像的明暗信息推断出物体表面几何形状的过程。这个问题假设光照条件已知,目标表面是光滑且均匀的,并且照明是单向的。其基本思想是根据目标表面对光照的反应,推断出表面法线,从而得到表面的三维形状。
1.1 渲染方程
渲染方程为:
L o ( x , ω o ) = L e ( x , ω o ) + ∫ Ω f ( x , ω i , ω o ) L i ( x , ω i ) ( n ⋅ ω i ) d ω i L_o(\mathbf{x}, \omega_o) = L_e(\mathbf{x}, \omega_o) + \int_{\Omega} f(\mathbf{x}, \omega_i, \omega_o) L_i(\mathbf{x}, \omega_i) (\mathbf{n} \cdot \omega_i) d\omega_i Lo(x,ωo)=Le(x,ωo)+∫Ωf(x,ωi,ωo)Li(x,ωi)(n⋅ωi)dωi
我们将其简化:
L
out
=
ρ
⋅
L
in
⋅
n
⊤
s
=
R
(
n
)
L_{\text{out}} = \rho \cdot L_{\text{in}} \cdot n^\top s = R(n)
Lout=ρ⋅Lin⋅n⊤s=R(n)
- L out L_{\text{out}} Lout :表示出射光线
- ρ \rho ρ :表示该点的漫反射率
- L in L_{\text{in}} Lin :表示入射光线
- n n n :表示表面法线
1.2 梯度空间表示法
梯度空间表示法 Gradient Space Representation,使用梯度信息来表示物体表面的几何形状。
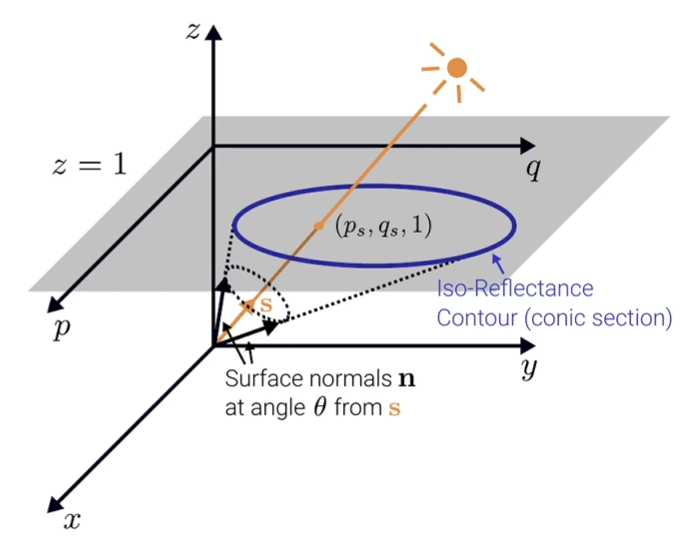
在梯度空间表示法中,给定光线 s s s 和观察到的反射率 R R R,我们可以通过以下方式来计算法线 n n n:
- 计算 n ⊤ s = cos ( θ ) n^\top s = \cos(\theta) n⊤s=cos(θ),得到 θ \theta θ —— 法线 n n n 与光线 s s s 之间的夹角
- 按照给定的角度 θ \theta θ,从光线 s s s 开始,投影这个集合到 z = 1 z = 1 z=1 平面上,我们可以得到一个锥面曲线即反射率曲线 Iso-Reflectance Contour,它表示出了所有与 s s s 的夹角为 θ \theta θ 的可能法线
通过不同的反射率 R R R ,我们可以得到反射率图(Reflectance Map):
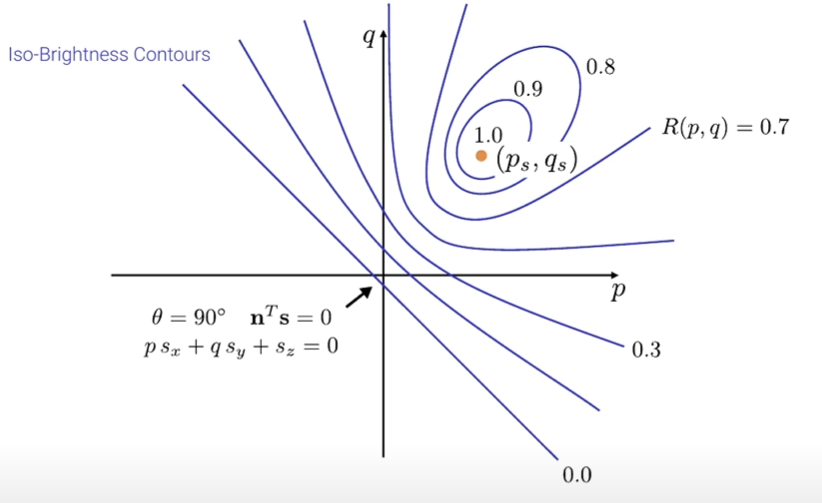
因此,在梯度空间表示法中,我们可以通过结合反射率图和其他几何信息来有效地参数化法线,并利用这些信息来推断物体表面的形状和材质。
2 光度立体法
光度立体法(Photometric Stereo)是通过在相同视点但采用不同(已知)点光源的多张图像来实现三维重建的。其需要对每个像素的法线和反射率进行估计。
2.1 采集 K 张图像
在相同的相机视点下,采集 K 张图像,每张图像使用不同的已知点光源。这些光源的位置和方向应该是已知的,并且在不同的图像中有所变化。
通过如下的反射率图可以看到,对于每个像素,我们必须要有3个反射率图才能确定一个真正的法线,所以,我们至少要有从3个不同的方向来的光源
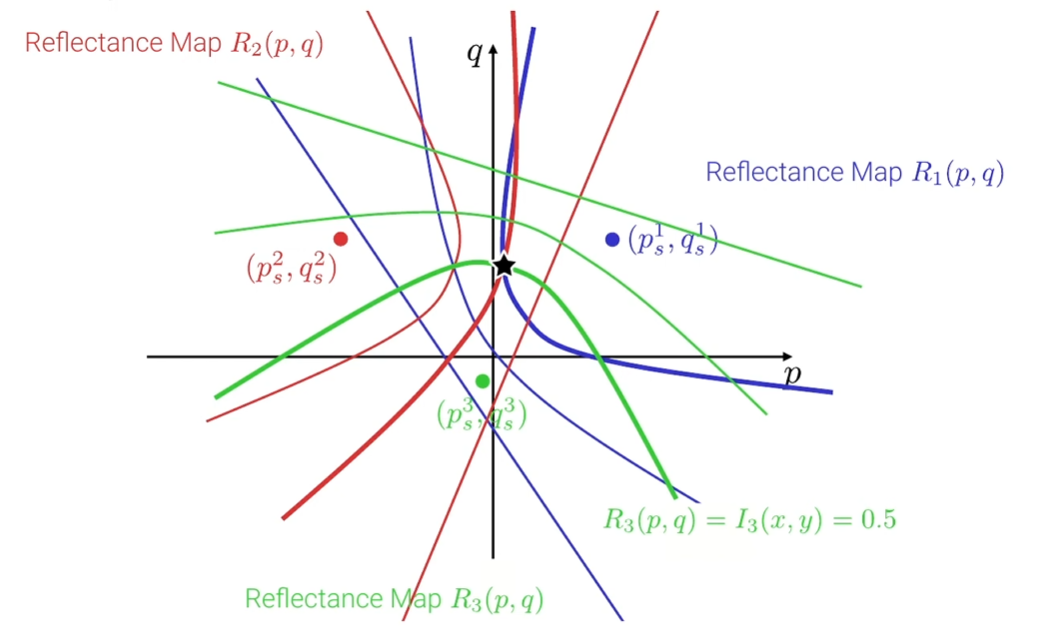
但是要避免共线光源:当光度立体设置中使用的所有光源都是共线时(位于同一直线或平面上),所得的线性系统将变得秩亏。这使得不可能唯一地确定每个像素的表面法线。因此,光度立体无法提供准确的重建。
2.2 光度法线与反射率估计
对于每个像素,利用 K 张图像中的光照信息,通过解光度立体方程组来估计法线和反射率:
使用兰伯反射,并且入射光强度为 L in = 1 L_{\text{in}} = 1 Lin=1,那么图像的亮度 I I I 可以表示为:
I = L out = ρ ⋅ n ⊤ s = ρ ⋅ s ⊤ n I = L_{\text{out}} = \rho \cdot n^\top s = \rho \cdot s^\top n I=Lout=ρ⋅n⊤s=ρ⋅s⊤n
对于给定的三个观测(相同的 v v v,不同的 s s s),我们可以将其表示为矩阵形式如下:
[ I 1 I 2 I 3 ] = [ s 1 ⊤ s 2 ⊤ s 3 ⊤ ] ⋅ ρ ⋅ n \begin{bmatrix} I_1 \\ I_2 \\ I_3 \end{bmatrix} = \begin{bmatrix} s_1^\top \\ s_2^\top \\ s_3^\top \end{bmatrix} \cdot \rho \cdot n I1I2I3 = s1⊤s2⊤s3⊤ ⋅ρ⋅n
其中, I 1 , I 2 , I 3 I_1, I_2, I_3 I1,I2,I3 分别是三个观测得到的图像亮度, s 1 , s 2 , s 3 s_1, s_2, s_3 s1,s2,s3 分别是对应的三个光源方向向量, ρ \rho ρ 是漫反射率, n n n 是法线向量。
通过使用更多的光源可以获得更好的结果(通过对测量进行平均)。通过最小二乘解法我们得到:
ρ n = ( S ⊤ S ) − 1 S ⊤ I \rho n = (S^\top S)^{-1}S^\top I ρn=(S⊤S)−1S⊤I
其中, S S S 是包含所有光源方向 s i s_i si 的矩阵, I I I 是包含对应图像亮度 I i I_i Ii 的向量
得到 ρ n \rho n ρn 后,可以通过 ρ = ∣ ∣ ρ n ∣ ∣ 2 \rho = ||\rho n||_2 ρ=∣∣ρn∣∣2 ( n n n 是单位向量 )来计算漫反射率 ρ \rho ρ , n = ρ n / ρ n = \rho n / \rho n=ρn/ρ
简单实现代码如下:
def compute_normals_albedo_map(imgs, mask, light_positions):
"""
imgs np.array [k,h,w] np.float32 [0.0, 1.0]
mask np.array [h,w] np.bool
light_positions np.array [k,3] np.float32
---
dims:
k: number of images
h: image height (num rows)
w: image width (num cols)
"""
S = light_positions
I = imgs.reshape(imgs.shape[0], -1)
# rho n = (S^T S)^-1 S^T I
rho_n = np.linalg.inv(S.T @ S) @ S.T @ I
# rho = ||rho_n||
rho = np.linalg.norm(rho_n, axis=0)
# n = rho_n / rho
n = np.divide(rho_n, rho, out=np.zeros_like(rho_n), where=rho != 0)
# mask out
mask_flat = mask.flatten()
n[:, ~mask_flat] = 0
normals_unit = n.T.reshape(imgs.shape[1], imgs.shape[2], 3)
rho = rho.reshape(imgs.shape[1], imgs.shape[2])
assert normals_unit.shape == (imgs.shape[1], imgs.shape[2], 3)
assert rho.shape == (imgs.shape[1], imgs.shape[2])
rho = np.clip(rho, 0.0, 1.0)
normals_unit = np.clip(normals_unit, 0.0, 1.0)
return normals_unit, rho, mask
输出是:
normals_unit
:三维数组,表示每个像素点的单位法线向量。它的形状是(imgs.shape[1], imgs.shape[2], 3)
,其中imgs.shape[1]
和imgs.shape[2]
分别是图像的高度和宽度,3
表示每个像素点的法线有三个分量(x、y、z)rho
:二维数组,表示每个像素点的漫反射率。它的形状是(imgs.shape[1], imgs.shape[2])
,与图像的大小相同,对应每一个像素的反射率
2.3 重新照亮场景
我们现在知道整个图像的像素法线和反照率,这使我们能够重新照亮场景(即人为地改变灯光位置)
def relight_scene(light_pos, normals_unit, albedo, mask):
"""
light_pos np.array [k,3] np.float32
mask np.array [h,w] np.bool
----
dims:
h: image height (num rows)
w: image width (num cols)
----
returns:
imgs np.array [h,w] np.float32 [0.0, 1.0]
"""
assert light_pos.shape == (3,)
assert np.allclose(1.0, np.linalg.norm(light_pos))
assert normals_unit.shape[-1] == 3
assert len(normals_unit.shape) == 3
img = albedo * (normals_unit @ light_pos)
# mask out
img[~mask] = 0
img_norm = np.clip(img, 0.0, 1.0)
assert np.all(
np.logical_and(0.0 <= img_norm, img_norm <= 1.0)
), "please normalize your image to interval [0.0,1.0]"
return img_norm
其接受灯光位置、像素法线、反射率和掩码作为输入,并返回重新照亮后的图像。
其实其中核心就是:根据之前的简化的渲染公式 L out = ρ ⋅ L in ⋅ n ⊤ s L_{\text{out}} = \rho \cdot L_{\text{in}} \cdot n^\top s Lout=ρ⋅Lin⋅n⊤s ,其中设置入射光线为1,即可得:
img = albedo * (normals_unit @ light_pos)