目录
摘要
基本原理
通道注意力机制
空间注意力机制
GAM代码实现
Wise-IoU
WIoU代码实现
yaml文件编写
完整代码分享(含多种注意力机制)
摘要
人们已经研究了各种注意力机制来提高各种计算机视觉任务的性能。然而,现有方法忽视了保留通道和空间方面的信息以增强跨维度交互的重要性。因此,我们提出了一种全局注意力机制,通过减少信息减少和放大全局交互表示来提高深度神经网络的性能。引入了具有多层感知器的 3D 排列,用于通道注意以及卷积空间注意子模块。在 CIFAR-100 和 ImageNet-1K 上对所提出的图像分类任务机制的评估表明,我们的方法稳定优于最近使用 ResNet 和轻量级 MobileNet 的几种注意力机制。
基本原理
目标的设计是一种减少信息缩减并放大全局维度交互特征的机制。我们采用 CBAM 的顺序通道空间注意力机制并重新设计子模块。整个过程如图 所示。
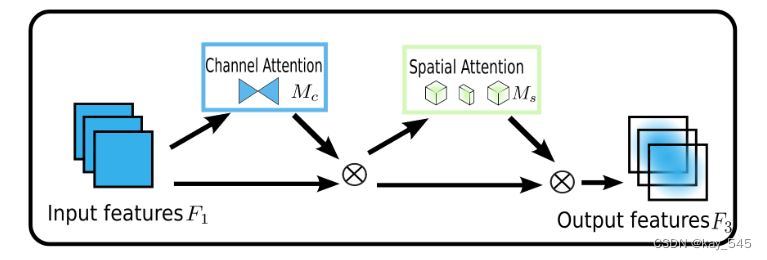
通道注意力机制
通道注意力子模块使用 3D 排列来保留三个维度的信息。然后,它使用两层 MLP(多层感知器)放大跨维度通道空间依赖性。 (MLP是一种编码器-解码器结构,其缩减比为r,与BAM相同。)通道注意子模块如图所示。
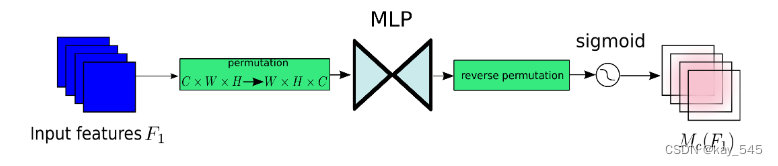
空间注意力机制
在空间注意力子模块中,为了关注空间信息,我们使用两个卷积层进行空间信息融合。我们还使用与 BAM 相同的通道注意子模块的缩减率 r。同时,最大池化会减少信息并产生负面影响。我们删除池化以进一步保留特征图。因此,空间注意力模块有时会显着增加参数的数量。为了防止参数显着增加,我们在 ResNet50 中采用带有通道洗牌的组卷积。没有组卷积的空间注意力子模块如图所示。
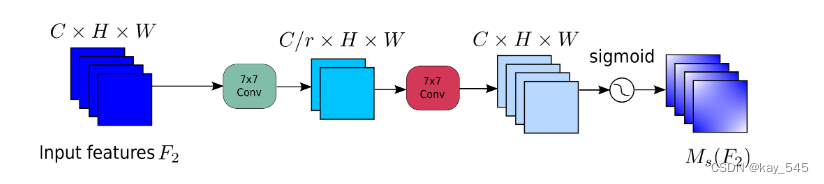
GAM代码实现
class GAM_Attention(nn.Module):
def __init__(self, c1, c2, group=True, rate=4):
super(GAM_Attention, self).__init__()
self.channel_attention = nn.Sequential(
nn.Linear(c1, int(c1 / rate)),
nn.ReLU(inplace=True),
nn.Linear(int(c1 / rate), c1)
)
self.spatial_attention = nn.Sequential(
nn.Conv2d(c1, c1 // rate, kernel_size=7, padding=3, groups=rate) if group else nn.Conv2d(c1, int(c1 / rate),
kernel_size=7,
padding=3),
nn.BatchNorm2d(int(c1 / rate)),
nn.ReLU(inplace=True),
nn.Conv2d(c1 // rate, c2, kernel_size=7, padding=3, groups=rate) if group else nn.Conv2d(int(c1 / rate), c2,
kernel_size=7,
padding=3),
nn.BatchNorm2d(c2)
)
def forward(self, x):
b, c, h, w = x.shape
x_permute = x.permute(0, 2, 3, 1).view(b, -1, c)
x_att_permute = self.channel_attention(x_permute).view(b, h, w, c)
x_channel_att = x_att_permute.permute(0, 3, 1, 2)
# x_channel_att=channel_shuffle(x_channel_att,4) #last shuffle
x = x * x_channel_att
x_spatial_att = self.spatial_attention(x).sigmoid()
x_spatial_att = channel_shuffle(x_spatial_att, 4) # last shuffle
out = x * x_spatial_att
# out=channel_shuffle(out,4) #last shuffle
return out
以上代码添加在 ./ultralytics/nn/modules/conv.py 中
Wise-IoU
Yolov7提出的损失函数是GIoU(Generalized Intersection over Union),能在更广义的层面上计算IoU(Intersection over Union),但是当两个预测框完全重合时,不能反映出实际情况,此时GIoU就要退化为IoU,并且GIoU对每个预测框与真实框均要计算最小外接框,故损失函数计算及收敛速度受到限制。
为了弥补这种遗憾,改进的网络中使用了WIoU(Wise-IoU)作为损失函数。WIoU v3作为边界框回归损失,包含一种动态非单调机制,并设计了一种合理的梯度增益分配,该策略减少了极端样本中出现的大梯度或有害梯度。该损失方法计算更多地关注普通质量的样本,进而提高网络模型的泛化能力和整体性能。
虽然几种主流损失函数都采用静态聚焦机制,但WIoU不仅考虑了方位角、质心距离和重叠面积,还引入了动态非单调聚焦机制。 WIoU应用合理的梯度增益分配策略来评估锚框的质量。WIoU有三个版本。 WIoU v1 设计了基于注意力的预测框损失,WIoU v2 和 WIoU v3 添加了聚焦系数。
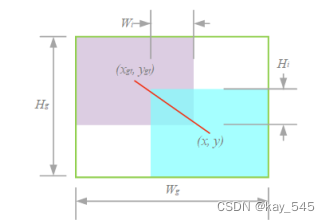
最小的包围盒(绿色)和中心点的连接(红色),其中并集的面积为 Su = wh + wgthgt − WiHi .
WIoU代码实现
def WIoU(cls, pred, target, self=None):
self = self if self else cls(pred, target)
dist = torch.exp(self.l2_center / self.l2_box.detach())
return self._scaled_loss(dist * self.iou)
下面的代码替换loss.py的class BboxLoss
class BboxLoss(nn.Module):
def __init__(self, reg_max, use_dfl=False):
"""Initialize the BboxLoss module with regularization maximum and DFL settings."""
super().__init__()
self.reg_max = reg_max
self.use_dfl = use_dfl
def forward(self, pred_dist, pred_bboxes, anchor_points, target_bboxes, target_scores, target_scores_sum, fg_mask):
"""IoU loss."""
weight = target_scores.sum(-1)[fg_mask].unsqueeze(-1)
loss,iou = bbox_iou(pred_bboxes[fg_mask], target_bboxes[fg_mask], xywh=False,type_='WIoU')
loss_iou=loss.sum()/target_scores_sum
# DFL loss
if self.use_dfl:
target_ltrb = bbox2dist(anchor_points, target_bboxes, self.reg_max)
loss_dfl = self._df_loss(pred_dist[fg_mask].view(-1, self.reg_max + 1), target_ltrb[fg_mask]) * weight
loss_dfl = loss_dfl.sum() / target_scores_sum
else:
loss_dfl = torch.tensor(0.0).to(pred_dist.device)
return loss_iou, loss_dfl
yaml文件编写
# Ultralytics YOLO 🚀, AGPL-3.0 license
# YOLOv8 object detection model with P3-P5 outputs. For Usage examples see https://docs.ultralytics.com/tasks/detect
# Parameters
nc: 1 # number of classes
scales: # model compound scaling constants, i.e. 'model=yolov8n.yaml' will call yolov8.yaml with scale 'n'
# [depth, width, max_channels]
n: [0.33, 0.25, 1024] # YOLOv8n summary: 225 layers, 3157200 parameters, 3157184 gradients, 8.9 GFLOPs
s: [0.33, 0.50, 1024] # YOLOv8s summary: 225 layers, 11166560 parameters, 11166544 gradients, 28.8 GFLOPs
m: [0.67, 0.75, 768] # YOLOv8m summary: 295 layers, 25902640 parameters, 25902624 gradients, 79.3 GFLOPs
l: [1.00, 1.00, 512] # YOLOv8l summary: 365 layers, 43691520 parameters, 43691504 gradients, 165.7 GFLOPs
x: [1.00, 1.25, 512] # YOLOv8x summary: 365 layers, 68229648 parameters, 68229632 gradients, 258.5 GFLOPs
# YOLOv8.0n backbone
backbone:
# [from, repeats, module, args]
- [-1, 1, Conv, [64, 3, 2]] # 0-P1/2
- [-1, 1, Conv, [128, 3, 2]] # 1-P2/4
- [-1, 3, C2f, [128, True]]
- [-1, 1, Conv, [256, 3, 2]] # 3-P3/8
- [-1, 6, C2f, [256, True]]
- [-1, 1, Conv, [512, 3, 2]] # 5-P4/16
- [-1, 6, C2f, [512, True]]
- [-1, 1, Conv, [1024, 3, 2]] # 7-P5/32
- [-1, 3, C2f, [1024, True]]
- [-1, 3, GAM_Attention, [1024]]
- [-1, 1, SPPF, [1024, 5]] # 10
# YOLOv8.0n head
head:
- [-1, 1, nn.Upsample, [None, 2, 'nearest']]
- [[-1, 6], 1, Concat, [1]] # cat backbone P4
- [-1, 3, C2f, [512]] # 13
#- [-1, 1, GAM_Attention, [512,512]]
- [-1, 1, nn.Upsample, [None, 2, 'nearest']]
- [[-1, 4], 1, Concat, [1]] # cat backbone P3
- [-1, 3, C2f, [256]] # 16 (P3/8-small)
#- [-1, 1, GAM_Attention, [256,256]]
- [-1, 1, Conv, [256, 3, 2]]
- [[-1, 13], 1, Concat, [1]] # cat head P4
- [-1, 3, C2f, [512]] # 19 (P4/16-medium)
#- [-1, 1, GAM_Attention, [512,512]]
- [-1, 1, Conv, [512, 3, 2]]
- [[-1, 10], 1, Concat, [1]] # cat head P5
- [-1, 3, C2f, [1024]] # 22 (P5/32-large)
#- [-1, 1, GAM_Attention, [1024,1024]]
- [[16, 19, 22], 1, Detect, [nc]] # Detect(P3, P4, P5)
完整代码分享(含多种注意力机制)
内涵SA,CBAM,GAM,ECA等多种注意力机制
链接: https://pan.baidu.com/s/1T9bVifTPCRMv2t7eREsuEw?pwd=nbrt 提取码: nbrt
报错解决办法
YOLOv8 | 添加注意力机制报错KeyError:已解决,详细步骤-CSDN博客