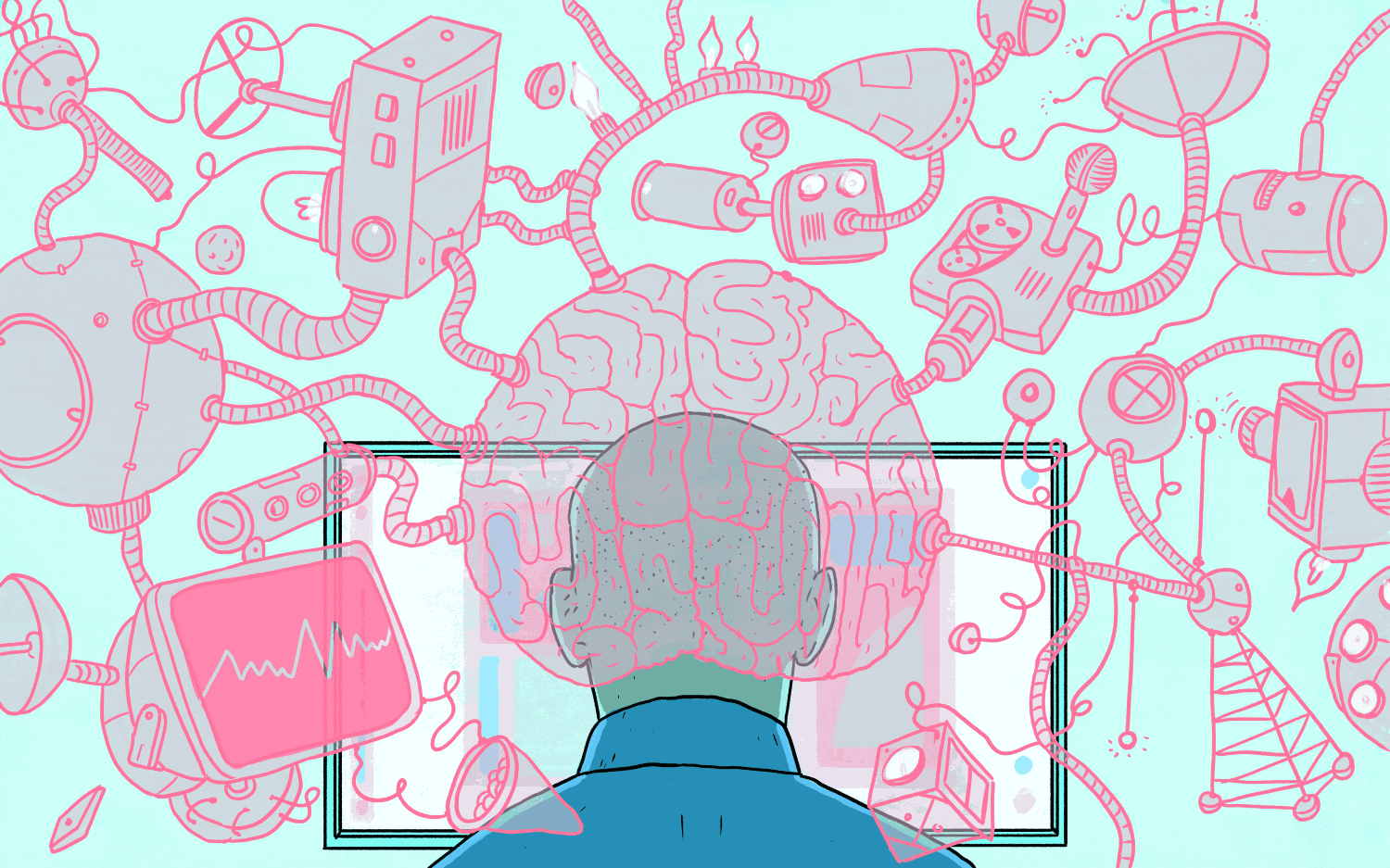
一、说明
本文 揭开CNN、Seq2Seq、Faster R-CNN 和 PPO — 编码和创新之路。对于此类编程的短小示例,用于开发时临时参考。
二、数据准备
问题陈述:在本次挑战中,您将深入计算机视觉世界并使用卷积神经网络 (CNN) 解决图像分类任务。您将使用 CIFAR-10 数据集,其中包含 10 个不同类别的 60,000 张不同图像。您的任务是构建一个 CNN 模型,能够准确地将这些图像分类为各自的类别。
# Image Classification with Convolutional Neural Networks (CNN)
import tensorflow as tf
from tensorflow.keras.datasets import cifar10
from tensorflow.keras.models import Sequential
from tensorflow.keras.layers import Conv2D, MaxPooling2D, Flatten, Dense
# Load the CIFAR-10 dataset
(x_train, y_train), (x_test, y_test) = cifar10.load_data()
# Preprocess the data
x_train, x_test = x_train / 255.0, x_test / 255.0
# Build a CNN model
model = Sequential([
Conv2D(32, (3, 3), activation='relu', input_shape=(32, 32, 3)),
MaxPooling2D((2, 2)),
Conv2D(64, (3, 3), activation='relu'),
MaxPooling2D((2, 2)),
Conv2D(64, (3, 3), activation='relu'),
Flatten(),
Dense(64, activation='relu'),
Dense(10)
])
# Compile the model
model.compile(optimizer='adam',
loss=tf.keras.losses.SparseCategoricalCrossentropy(from_logits=True),
metrics=['accuracy'])
# Train the model
model.fit(x_train, y_train, epochs=10, validation_data=(x_test, y_test))
二、用于机器翻译的序列到序列 (Seq2Seq) 模型
问题陈述:机器翻译在打破语言障碍、促进全球交流方面发挥着至关重要的作用。在本次挑战中,您将踏上自然语言处理 (NLP) 和深度学习之旅,以实现机器翻译的序列到序列 (Seq2Seq) 模型。您的任务是建立一个模型,可以有效地将文本从一种语言翻译成另一种语言。
# Sequence-to-Sequence (Seq2Seq) Model for Machine Translation
import tensorflow as tf
import numpy as np
from tensorflow.keras.models import Model
from tensorflow.keras.layers import Input, LSTM, Dense
# Define the encoder-decoder model for machine translation
latent_dim = 256
encoder_inputs = Input(shape=(None, num_encoder_tokens))
encoder_lstm = LSTM(latent_dim, return_state=True)
encoder_outputs, state_h, state_c = encoder_lstm(encoder_inputs)
encoder_states = [state_h, state_c]
decoder_inputs = Input(shape=(None, num_decoder_tokens))
decoder_lstm = LSTM(latent_dim, return_sequences=True, return_state=True)
decoder_outputs, _, _ = decoder_lstm(decoder_inputs, initial_state=encoder_states)
decoder_dense = Dense(num_decoder_tokens, activation='softmax')
decoder_outputs = decoder_dense(decoder_outputs)
model = Model([encoder_inputs, decoder_inputs], decoder_outputs)
# Compile and train the model for machine translation
model.compile(optimizer='adam', loss='categorical_crossentropy', metrics=['accuracy'])
model.fit([encoder_input_data, decoder_input_data], decoder_target_data, batch_size=batch_size, epochs=epochs, validation_split=0.2)
三、使用 Faster R-CNN 进行物体检测
问题陈述:您的任务是使用 Faster R-CNN(基于区域的卷积神经网络)模型实现对象检测。给定图像,您的目标是识别和定位图像中的对象,提供对象的类和边界框坐标。
# Object Detection with Faster R-CNN
import tensorflow as tf
from tensorflow.keras.applications import ResNet50
from tensorflow.keras.layers import Input
from tensorflow.keras.models import Model
from tensorflow.keras.models import load_model
from tensorflow.keras.preprocessing import image
from tensorflow.keras.applications.imagenet_utils import decode_predictions
# Load a pre-trained ResNet50 model
base_model = ResNet50(weights='imagenet')
# Add custom layers for object detection
x = base_model.layers[-2].output
output = Dense(num_classes, activation='softmax')(x)
model = Model(inputs=base_model.input, outputs=output)
# Load and preprocess an image for object detection
img_path = 'image.jpg'
img = image.load_img(img_path, target_size=(224, 224))
img = image.img_to_array(img)
img = np.expand_dims(img, axis=0)
img = tf.keras.applications.resnet.preprocess_input(img)
# Make predictions for object detection
preds = model.predict(img)
predictions = decode_predictions(preds, top=5)[0]
print(predictions)
四、使用近端策略优化 (PPO) 的强化学习
问题陈述:您正在进入强化学习 (RL) 的世界,并负责实施近端策略优化 (PPO) 算法来训练代理。使用 OpenAI Gym 的 CartPole-v1 环境,您的目标是开发一个 RL 代理,通过采取最大化累积奖励的行动来学习平衡移动推车上的杆子。
# Reinforcement Learning with Proximal Policy Optimization (PPO)
import gym
import tensorflow as tf
from tensorflow import keras
from tensorflow.keras.layers import Dense
# Create a Gym environment
env = gym.make('CartPole-v1')
# Build a PPO agent
model = keras.Sequential([
Dense(64, activation='relu', input_shape=(env.observation_space.shape[0],)),
Dense(32, activation='relu'),
Dense(env.action_space.n, activation='softmax')
])
optimizer = keras.optimizers.Adam(learning_rate=0.001)
model.compile(optimizer, loss='categorical_crossentropy')
# Train the agent using PPO
for episode in range(1000):
state = env.reset()
done = False
while not done:
action_probs = model.predict(state.reshape(1, -1))[0]
action = np.random.choice(env.action_space.n, p=action_probs)
next_state, reward, done, _ = env.step(action)
# Update the agent's policy using PPO training
# (Implementing PPO training is a more complex task)
state = next_state
关注AI更多资讯!旅程 — AI :Jasmin Bharadiya